AI And The "Poop" Podcast: Efficiently Processing Repetitive Scatological Data
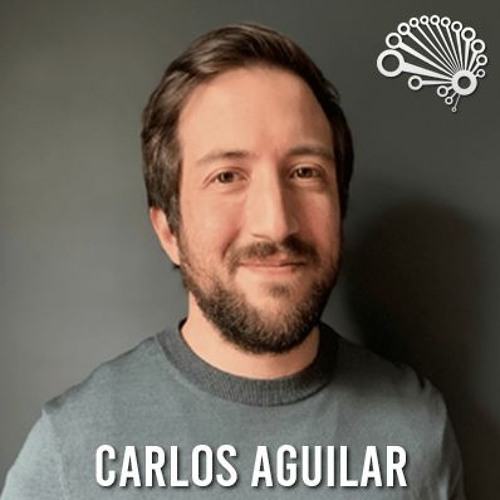
Table of Contents
Challenges of Manual "Poop" Podcast Data Analysis
Manually analyzing the data from numerous "poop" podcasts presents significant hurdles. The sheer volume and repetitive nature of the information, coupled with inconsistencies and inherent subjectivity, make manual analysis incredibly inefficient and prone to error.
Volume and Repetition
The sheer volume of data generated by the growing number of podcasts focusing on bowel movements and digestive health is overwhelming.
- Hours of audio to transcribe: Each podcast episode requires manual transcription, a process consuming significant time and resources.
- Countless mentions of similar symptoms: Many podcasts cover similar symptoms, leading to repetitive data entry and analysis.
- Difficulty identifying trends manually: Without AI assistance, spotting significant trends and patterns within the vast amount of scatological data is extremely challenging.
Data Inconsistency
Variations in terminology and descriptions of bowel movements across different podcasts significantly hinder consistent data analysis.
- Different terminology for bowel consistency: Podcasters may use varying terms to describe stool consistency (e.g., "loose," "soft," "formed"), making it difficult to standardize the data.
- Subjective descriptions varying between hosts and guests: The subjective nature of descriptions further complicates analysis, introducing inconsistencies and biases.
- Difficulty standardizing data for comparison: Without a standardized approach, comparing data across different podcasts becomes almost impossible.
Subjectivity and Bias
Manual analysis is inherently susceptible to subjective bias, compromising the reliability of the findings.
- Analyst's personal interpretations of data: The analyst's preconceived notions or interpretations can influence the analysis, leading to skewed results.
- Potential for overlooking crucial details: The sheer volume of information can lead to human error, potentially causing crucial details to be overlooked.
- Inconsistencies across multiple analysts: Different analysts might interpret the same data differently, introducing further inconsistencies in the findings.
AI-Powered Solutions for Efficient "Poop" Podcast Data Processing
Fortunately, AI offers a powerful solution to streamline the analysis of this repetitive scatological data. Several AI-powered tools and techniques can significantly improve efficiency and accuracy.
Automated Transcription and Summarization
AI can drastically reduce the manual workload associated with podcast analysis through automated transcription and summarization.
- Speech-to-text software integrated with natural language processing (NLP): Advanced AI tools can accurately transcribe audio podcast episodes into text, eliminating the need for manual transcription.
- AI identifying key topics and summarizing lengthy discussions: NLP algorithms can identify key themes and summarize lengthy discussions, significantly reducing review time.
- Generation of concise summaries for quick review: AI can generate concise summaries, allowing researchers to quickly grasp the essence of each episode.
Sentiment Analysis and Topic Modeling
AI algorithms can perform sentiment analysis to gauge the emotional tone of discussions and utilize topic modeling to identify recurring themes within the data.
- Identifying positive or negative sentiments related to specific bowel issues: AI can analyze listener sentiment towards specific digestive issues, providing valuable insights into public perception.
- Clustering similar discussions into topic models: Topic modeling helps group similar discussions together, revealing prevalent themes and trends related to digestive health.
- Revealing prevalent themes and trends related to digestive health: Identifying these patterns can inform podcast content and medical research.
Data Cleaning and Standardization
AI can play a critical role in cleaning and standardizing the inconsistent terminology frequently encountered in "poop" podcast data.
- Using NLP to identify synonyms and standardize terms related to bowel movements: AI can resolve inconsistencies in terminology, ensuring uniformity across different datasets.
- Resolving inconsistencies in data formats: AI can handle variations in data formatting, preparing the data for efficient analysis.
- Improving the reliability and comparability of data sets: Standardized data improves the accuracy and comparability of research findings.
Unlocking Valuable Insights with AI
By leveraging AI, researchers and podcasters can uncover valuable insights that would be impossible to obtain through manual analysis alone.
Identifying Trends and Patterns
AI can reveal hidden trends and patterns in scatological data, leading to a deeper understanding of digestive health.
- Identifying correlations between dietary habits and bowel movements: AI can analyze relationships between diet and bowel function, providing valuable insights for dietary recommendations.
- Discovering common symptoms associated with specific conditions: AI can help identify symptom clusters associated with various digestive disorders.
- Understanding the effectiveness of various treatments: AI can analyze the effectiveness of various treatments and interventions based on listener experiences.
Improving Podcast Content Strategy
Analyzing listener engagement and common themes can significantly improve podcast content strategy.
- Identifying popular topics for future episodes: AI can reveal popular topics, ensuring content resonates with the target audience.
- Tailoring content to address listener interests: By understanding listener preferences, podcasters can create more engaging and relevant content.
- Improving the quality and relevance of podcasts: Data-driven insights can lead to higher quality podcasts that better serve the audience.
Supporting Medical Research
Aggregated and analyzed data from "poop" podcasts can significantly support medical research on digestive health.
- Providing large datasets for epidemiological studies: AI-processed data can provide valuable datasets for large-scale epidemiological studies.
- Supporting the development of new diagnostic tools and treatments: Insights from data analysis can contribute to the innovation of new diagnostic tools and treatments.
- Contributing to a better understanding of digestive health: Ultimately, AI-driven analysis helps advance knowledge and improve the treatment of digestive conditions.
Conclusion
AI offers a powerful solution for efficiently processing the repetitive scatological data generated by the burgeoning "poop" podcast industry. By automating transcription, performing sentiment analysis, and standardizing data, AI enables researchers, podcasters, and healthcare professionals to unlock valuable insights into digestive health. This technology significantly reduces the time and effort required for data analysis, allowing for a more efficient and effective understanding of this important topic. Embrace the power of AI to optimize your "poop" podcast data analysis and discover new insights today!
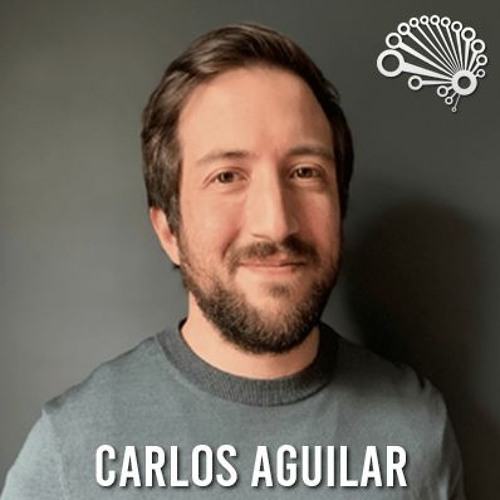
Featured Posts
-
Nadal Bids Tearful Adieu To Roland Garros Sabalenka Claims Victory
May 28, 2025 -
One Pieces Most Brutal Pirate Crews Their Crimes And Impact
May 28, 2025 -
Sinners Straight Sets Win Over Rinderknech At Roland Garros 2025
May 28, 2025 -
Nine Points 99th Minute Heartbreak How Ajax Lost The Championship
May 28, 2025 -
Thuc Hu Kho Bau 13 Trieu Usd Rau Den Dieu Tra Va Phan Tich Cac Bang Chung
May 28, 2025