AI-Driven Podcast Creation: Analyzing Repetitive Scatological Documents
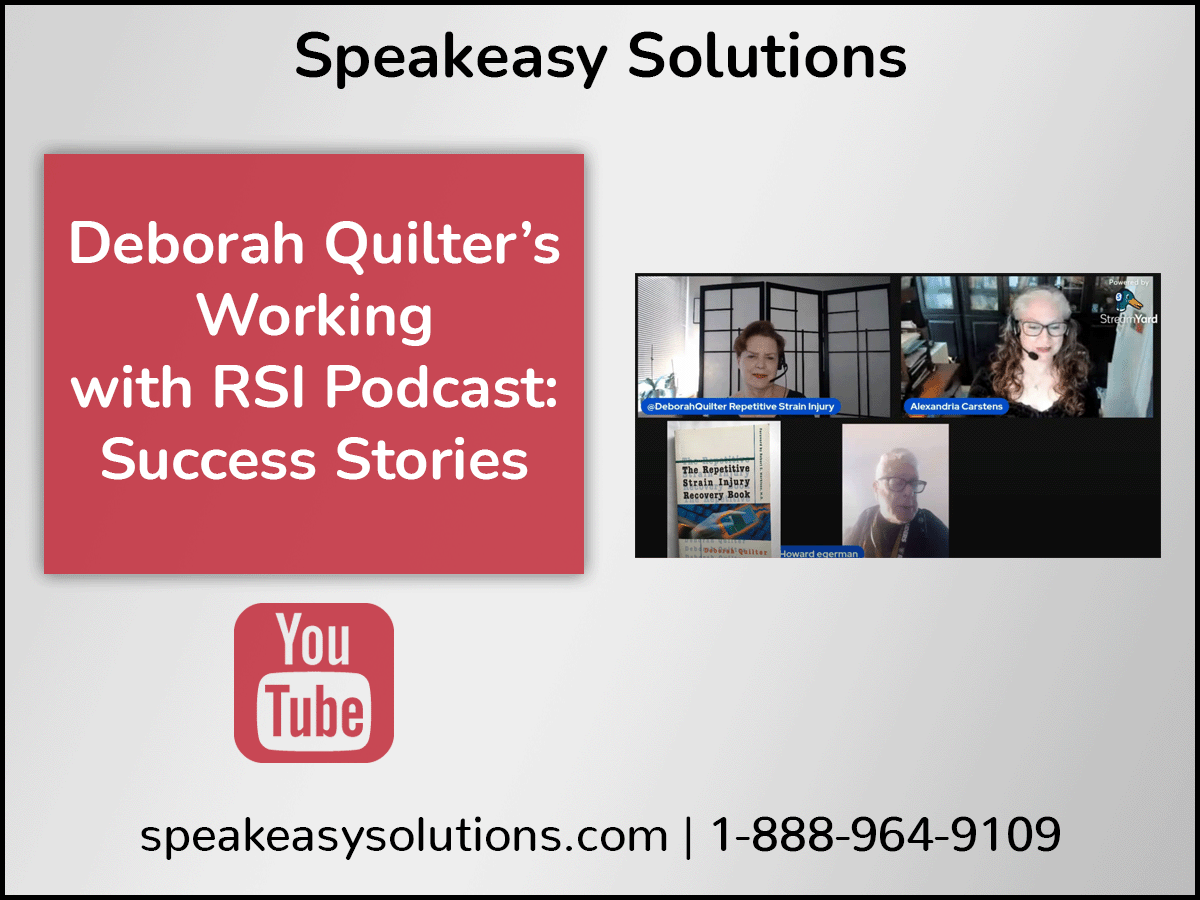
Table of Contents
Data Acquisition and Preprocessing
Before AI can weave its magic, we need the raw material: the scatological documents themselves. This stage, crucial for successful AI-driven podcast creation analyzing repetitive scatological documents, involves careful planning and execution.
Identifying and Gathering Relevant Documents
The first step is identifying and gathering the relevant documents. This might involve:
- Digital archives: Searching online repositories and digital libraries for digitized scatological documents.
- Online databases: Utilizing specialized databases containing relevant information, potentially requiring specific search queries and filters.
- Manual collection: In some cases, manual collection might be necessary, requiring careful handling and documentation of physical documents.
However, challenges abound:
- Data format inconsistencies: Documents might come in various formats (PDF, Word, images), requiring conversion and standardization.
- Missing data: Incomplete or fragmented documents will need careful handling or exclusion.
- Noisy data: Irrelevant information or errors within the documents need to be identified and removed.
Therefore, robust data cleaning and preprocessing are essential.
Data Cleaning and Preparation for AI Analysis
Raw scatological data is rarely ready for direct AI analysis. Thorough cleaning and preparation are paramount:
- Removing irrelevant information: This might include extraneous text, headers, footers, or irrelevant sections within the documents.
- Handling missing values: Missing data points can be imputed using various statistical techniques or removed if the impact is minimal.
- Converting data into a suitable format: The data needs to be structured appropriately for the chosen AI algorithms, often involving text extraction and formatting.
Specific text preprocessing techniques are vital:
- Stemming: Reducing words to their root forms (e.g., "running" to "run").
- Lemmatization: Reducing words to their dictionary form (lemma), considering context (e.g., "better" to "good").
- Stop word removal: Eliminating common words (e.g., "the," "a," "is") that don't contribute significantly to analysis.
AI-Driven Analysis Techniques
With the data prepared, we can leverage the power of AI. AI-driven podcast creation analyzing repetitive scatological documents relies heavily on advanced analytical techniques.
Topic Modeling and Sentiment Analysis
To understand the content of the scatological documents, we utilize:
- Topic Modeling: Algorithms like Latent Dirichlet Allocation (LDA) can identify recurring themes and patterns within the text. This allows us to group related information and uncover overarching narratives.
- Sentiment Analysis: This technique reveals the emotional tone and biases present in the documents, providing crucial context and insights. Various sentiment analysis libraries (e.g., NLTK, VADER) can be employed.
These techniques unveil hidden insights that might be missed through manual review:
- Uncovering recurring motifs: Identifying common themes and patterns in seemingly disparate documents.
- Identifying emotional shifts: Tracking changes in sentiment over time or across different sections of the data.
Named Entity Recognition (NER) and Relationship Extraction
Further enriching the analysis:
- Named Entity Recognition (NER): This identifies and classifies key entities within the text, such as people, places, organizations, dates, and quantities. This is particularly useful for identifying potential authors or sources of the documents.
- Relationship Extraction: This goes a step further, identifying the relationships between these entities. For instance, it might reveal who wrote which document or which organization was involved in a specific event.
This provides context and deeper understanding:
- Connecting seemingly unrelated documents: Revealing links between documents based on shared entities and relationships.
- Building a richer narrative: Providing more context and detail for the podcast's storyline.
Podcast Creation and Storytelling
The final stage translates the AI-generated insights into a compelling podcast. Effective AI-driven podcast creation analyzing repetitive scatological documents requires careful narrative design.
Structuring the Podcast Narrative
Transforming AI-generated data into a compelling narrative requires skill:
- Clear storytelling techniques: The podcast needs a clear beginning, middle, and end, engaging the listener from start to finish.
- Audience engagement: Consider using various storytelling techniques to maintain listener interest, like suspense, cliffhangers, or personal anecdotes.
Structuring elements include:
- Chronological approach: Presenting information in chronological order.
- Thematic organization: Grouping information based on identified topics or themes.
Using AI for Voice Synthesis and Audio Editing
AI further streamlines podcast production:
- AI-powered voice synthesis: Platforms like Amazon Polly or Google Cloud Text-to-Speech can generate natural-sounding voices to narrate the podcast.
- AI-driven audio editing: AI tools can automate tasks like noise reduction, sound equalization, and music selection, saving time and improving audio quality.
This results in a polished, professional product:
- Consistent and engaging audio: High-quality audio significantly enhances the listener experience.
- Efficient workflow: AI automates many time-consuming tasks, freeing up time for creative storytelling.
Conclusion
AI-driven podcast creation offers a powerful approach to analyzing repetitive scatological documents. By combining data acquisition, sophisticated AI analysis techniques, and innovative podcast production methods, researchers can uncover hidden narratives and insights previously buried in mountains of data. This methodology offers efficiency gains and deeper understanding. Unlock the power of AI to transform your repetitive scatological document analysis. Start exploring AI-driven podcast creation today and uncover hidden narratives!
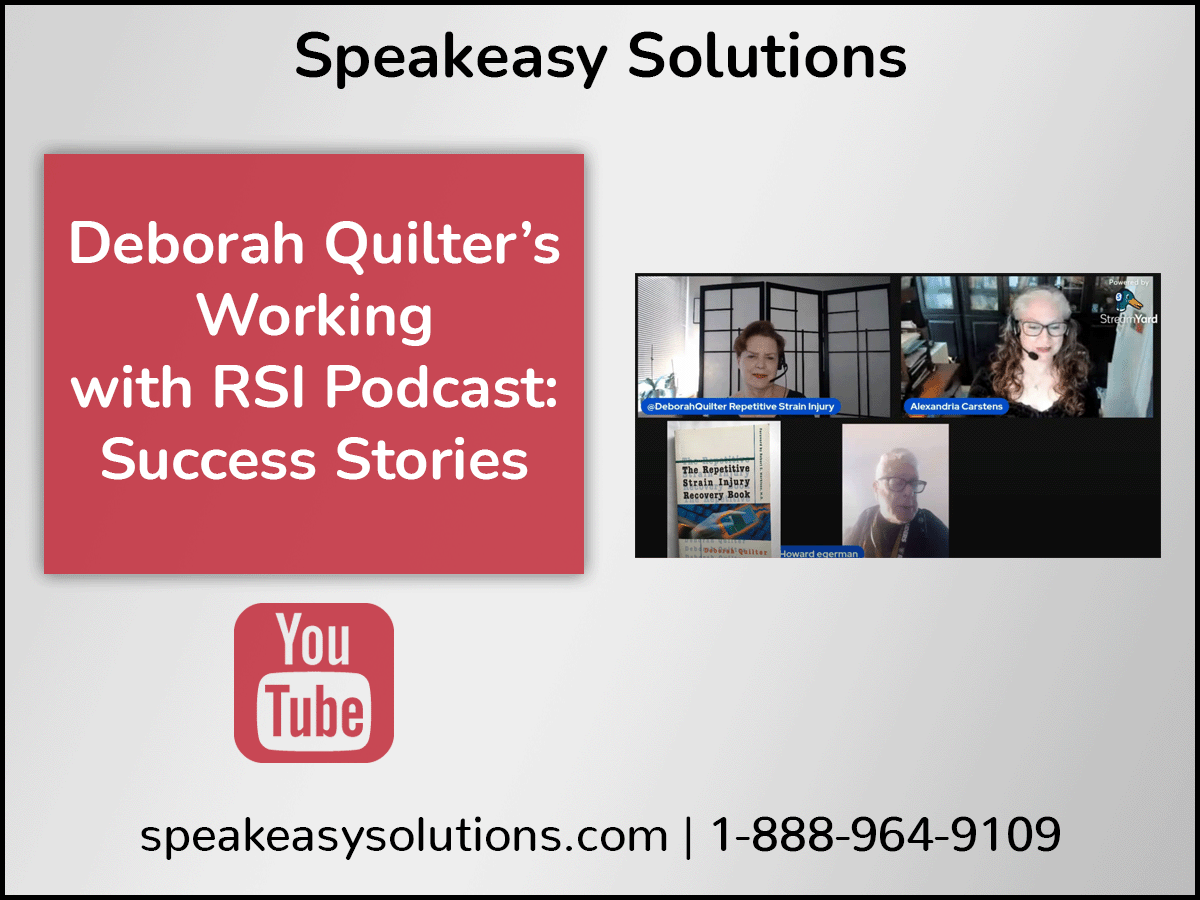
Featured Posts
-
Nyt Spelling Bee February 10 2025 Clues Answers And Spangram
Apr 29, 2025 -
Federal Investigation Hacker Made Millions Targeting Executive Office365 Accounts
Apr 29, 2025 -
Regulatory Green Light For Hengrui Pharmas Hong Kong Listing
Apr 29, 2025 -
The Russian Militarys Moves Keeping Europe On Edge
Apr 29, 2025 -
Premier League On The Verge Of Securing A Fifth Champions League Place
Apr 29, 2025
Latest Posts
-
The D C Black Hawk Crash A Comprehensive Report On Pilot Error
Apr 29, 2025 -
Emergency Response Downtown Louisville Buildings Evacuated Following Gas Leak
Apr 29, 2025 -
Analysis Of The Black Hawk Crash In D C Pilots Actions Under Scrutiny
Apr 29, 2025 -
Louisville Downtown Buildings Evacuated Due To Gas Leak Investigation
Apr 29, 2025 -
67 Lives Lost The Black Hawk Crash And The Pilots Fatal Decision
Apr 29, 2025