AI's Cognitive Abilities: What We Know And What We Don't
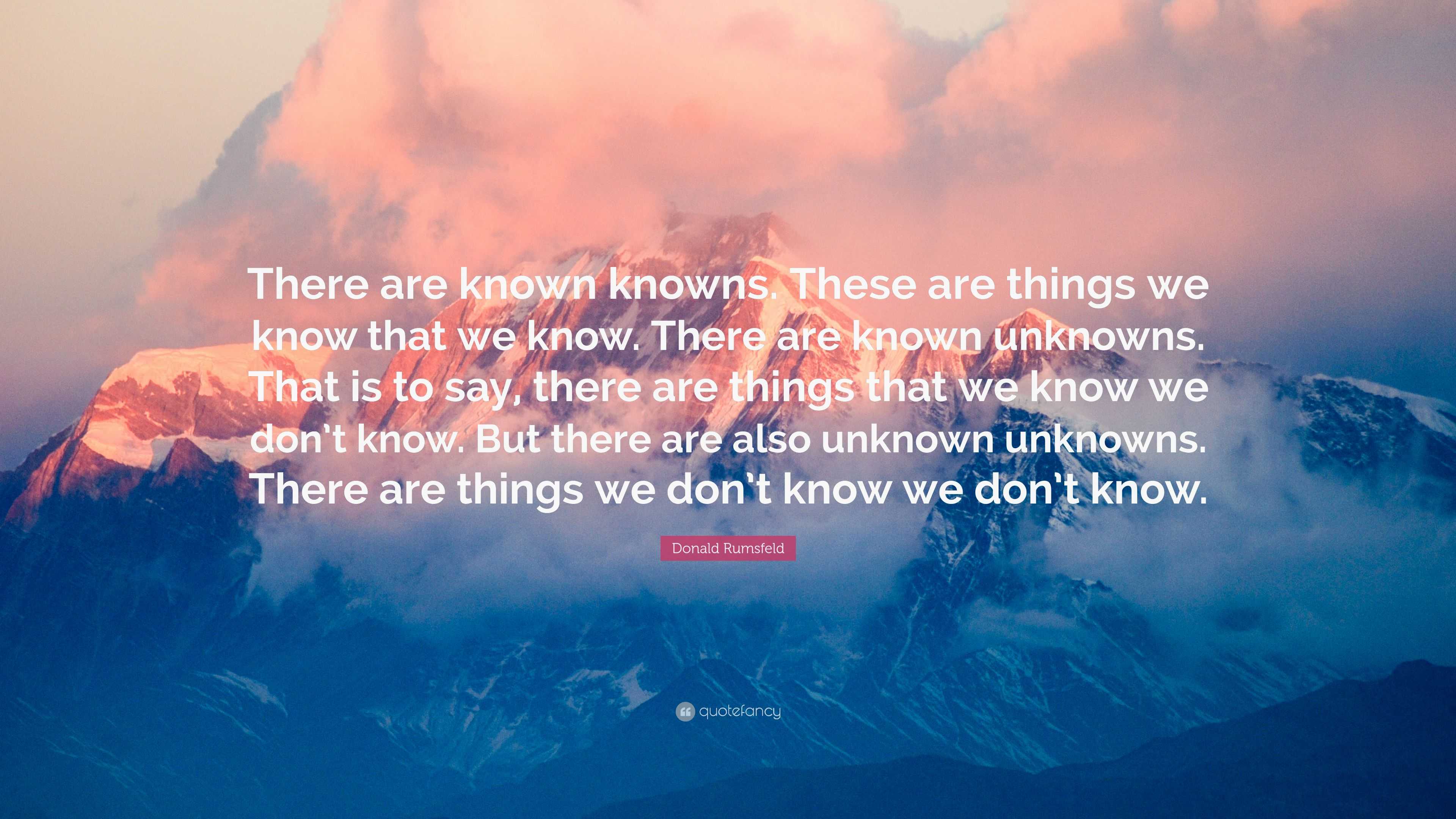
Table of Contents
Current Achievements in AI's Cognitive Abilities
AI's cognitive progress is undeniable, with several areas showcasing impressive capabilities.
Natural Language Processing (NLP)
Natural Language Processing (NLP) focuses on enabling computers to understand, interpret, and generate human language. This crucial aspect of AI's cognitive abilities is rapidly evolving.
- Examples: NLP powers chatbots like Siri and Alexa, facilitates real-time language translation services like Google Translate, and enables sentiment analysis for market research and social media monitoring.
- Limitations: Despite advancements, NLP struggles with nuances like sarcasm, humor, and understanding context fully. Ambiguity in language remains a significant hurdle.
- Future potential: We can expect more sophisticated dialogue systems capable of nuanced conversations, and significant improvements in machine translation accuracy, reducing reliance on human intervention. The development of truly multilingual AI systems is also a key area of ongoing research within NLP.
Computer Vision
Computer vision grants AI the capacity to "see" and interpret images and videos, mimicking human visual perception.
- Examples: Object recognition in self-driving cars, facial recognition for security purposes, and medical image analysis for disease detection are all powered by computer vision.
- Limitations: While impressive, computer vision algorithms still struggle with complex scenes, inconsistent lighting conditions, and situations where objects are occluded. The ability to understand the context of images and videos remains a challenge.
- Future potential: The development of autonomous vehicles relies heavily on advancements in computer vision. Furthermore, improved medical diagnostics and enhanced surveillance technologies are expected as computer vision capabilities continue to develop. The creation of robust visual scene understanding systems is a key future goal.
Problem-Solving and Reasoning
AI's ability to solve problems and draw logical conclusions demonstrates growing sophistication in its cognitive skills.
- Examples: AI excels at game playing, famously defeating human champions in chess and Go. Expert systems are used in various fields, offering diagnoses and solutions in areas like medicine and finance.
- Limitations: While impressive in specific domains, AI often struggles with abstract reasoning and common-sense knowledge. The ability to adapt learned knowledge to completely new situations remains a major challenge.
- Future potential: More robust decision-making systems for complex situations are expected, leading to improvements in scientific discovery, financial modeling, and resource management. The integration of common-sense reasoning into AI systems remains a key research area.
The Unknowns and Limitations of AI's Cognitive Abilities
Despite significant progress, considerable unknowns and limitations persist in the realm of AI's cognitive abilities.
Consciousness and Sentience
The question of whether AI can achieve genuine consciousness or sentience remains a hotly debated topic.
- Discussion Points: The philosophical debate surrounding machine consciousness involves the Turing Test and other measures of intelligence. The very definition of consciousness remains elusive.
- Open Questions: Can AI truly understand and feel emotions? What are the ethical implications of creating truly conscious machines? These are critical questions that require ongoing discussion and careful consideration.
Generalization and Transfer Learning
AI models often struggle to generalize knowledge gained from one task to another.
- Explanation: An AI trained to recognize cats might not be able to recognize dogs without extensive retraining. This lack of transfer learning limits its adaptability.
- Research Areas: Active research focuses on transfer learning, domain adaptation, and lifelong learning – enabling AI to learn continuously and apply knowledge across diverse contexts.
Bias and Fairness in AI
Data used to train AI models often contains inherent biases, leading to unfair or discriminatory outcomes.
- Examples: Bias in facial recognition systems disproportionately misidentifies individuals from certain ethnic groups. Biased datasets can lead to unfair loan applications or discriminatory hiring practices.
- Solutions: Developing methods for detecting and mitigating bias in AI algorithms is crucial for ensuring fairness and equity. This involves careful data curation and the development of bias-aware algorithms.
The Future of AI's Cognitive Abilities
The future of AI's cognitive abilities promises exciting advancements.
Explainable AI (XAI)
The need for greater transparency and understanding in AI decision-making is driving the development of Explainable AI (XAI).
- Importance: Building trust and accountability in AI systems is paramount, especially in high-stakes applications like healthcare and finance.
- Research Focus: Developing techniques to make AI models more interpretable and understandable is a major research focus.
Neuromorphic Computing
Neuromorphic computing aims to develop hardware that mimics the structure and function of the human brain.
- Potential: This could lead to AI systems with greater energy efficiency and potentially more advanced cognitive capabilities.
- Challenges: Significant engineering and technological hurdles need to be overcome to realize the full potential of neuromorphic computing.
Hybrid AI Systems
Combining different AI approaches can leverage complementary strengths for improved performance.
- Examples: Combining symbolic AI with machine learning can create more robust and adaptable systems.
- Benefits: Hybrid approaches can address limitations of individual AI methods, leading to more powerful and versatile systems.
Conclusion
AI's cognitive abilities are rapidly evolving, demonstrating impressive achievements in various domains. However, significant limitations remain, particularly concerning consciousness, generalization, and bias. Continued research in Explainable AI, neuromorphic computing, and hybrid AI systems is essential for unlocking the full potential of AI while mitigating its risks. Understanding both the advancements and limitations of AI's cognitive abilities is crucial for responsible innovation and deployment. To stay informed about the latest developments and ethical considerations, continue exploring resources on AI's cognitive abilities and related fields.
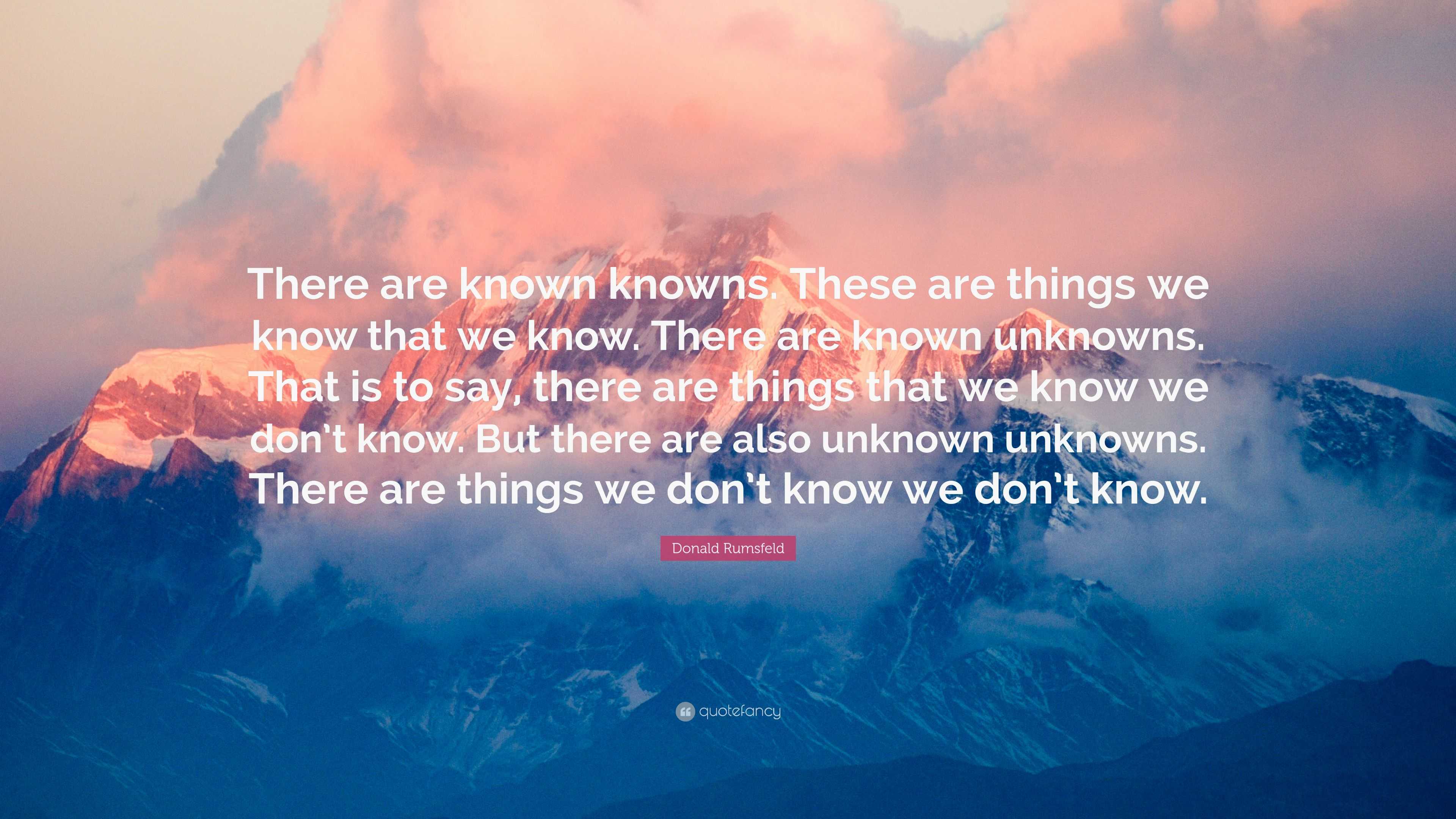
Featured Posts
-
Merd Fn Abwzby 2024 Kl Ma Thtaj Merfth
Apr 29, 2025 -
End Of Ryujinx Emulator Development Ceases After Nintendo Intervention
Apr 29, 2025 -
Bof A On Stock Market Valuations Why Investors Shouldnt Panic
Apr 29, 2025 -
Analyzing The Surge Of Vacant Office Buildings In Chicago
Apr 29, 2025 -
Understanding High Stock Market Valuations A Bof A Analysis
Apr 29, 2025
Latest Posts
-
Crooks Office365 Hacking Spree Yields Millions Federal Charges Allege
Apr 29, 2025 -
Office365 Executive Accounts Targeted In Multi Million Dollar Hacking Scheme
Apr 29, 2025 -
Federal Investigation Hacker Made Millions Targeting Executive Office365 Accounts
Apr 29, 2025 -
Millions Stolen In Exec Office365 Account Compromise Federal Charges Filed
Apr 29, 2025 -
Office365 Intrusion Nets Millions For Hacker Fbi Investigation Reveals
Apr 29, 2025