Ensuring Accurate Results: A Robust Poll Data System
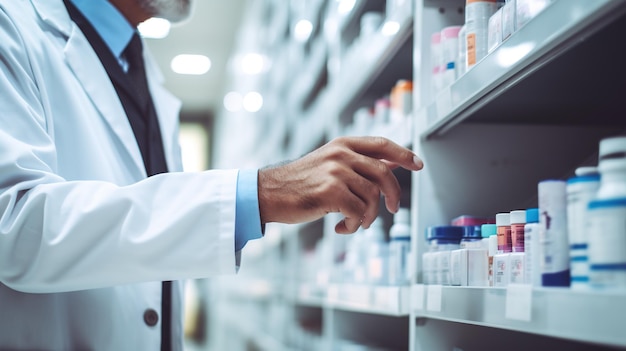
Data Collection Methodology: The Foundation of Accuracy
The accuracy of your poll data hinges on a well-defined sampling method. A poorly designed sampling strategy can introduce significant bias, rendering your results meaningless. Choosing the right approach is paramount for building a robust poll data system.
Different sampling techniques offer various advantages and disadvantages. Understanding these nuances is key to selecting the most appropriate method for your specific needs.
- Random Sampling: Every member of the population has an equal chance of being selected. This method is simple to implement but can be inefficient if the population is diverse.
- Stratified Sampling: The population is divided into subgroups (strata), and a random sample is taken from each stratum. This ensures representation from all subgroups, improving accuracy, especially when dealing with diverse populations.
- Cluster Sampling: The population is divided into clusters, and a random sample of clusters is selected. All members within the selected clusters are then included in the sample. This method is cost-effective for large, geographically dispersed populations but may increase sampling error.
Here's a breakdown of crucial considerations for data collection:
- Advantages and Disadvantages of Each Sampling Technique: Random sampling is easy but may miss subgroups; stratified sampling is accurate for diverse populations but requires careful stratification; cluster sampling is cost-effective but may have higher sampling error.
- Sample Size Calculation and its Impact on Accuracy: A larger sample size generally leads to greater accuracy, but it's crucial to calculate the appropriate sample size based on the desired margin of error and confidence level. Using online sample size calculators can be helpful.
- Minimizing Sampling Bias through Careful Planning: Careful planning, including defining the target population precisely and using appropriate sampling techniques, helps minimize bias.
- Addressing Potential Non-response Bias: Non-response bias arises when those who don't respond differ systematically from those who do. Strategies to mitigate this include follow-up attempts and weighting techniques.
Secure Data Storage and Management
Data security and privacy are paramount in any robust poll data system. Protecting sensitive respondent information is not only ethically responsible but also legally mandated in many jurisdictions. Compromised data can damage your reputation and lead to legal repercussions.
Several data storage options exist, each with its own security implications:
- Cloud Storage: Offers scalability and accessibility but relies on the security measures of the cloud provider. Careful selection of a reputable provider with strong security protocols is essential.
- Local Servers: Offer greater control over data security but require significant investment in infrastructure and maintenance.
Key elements of secure data management include:
- Data Encryption: Encrypting data both in transit and at rest safeguards against unauthorized access.
- Regular Data Backups and Disaster Recovery Plans: Regular backups and a robust disaster recovery plan ensure data availability even in the event of a system failure or cyberattack.
- Compliance with Data Privacy Regulations (GDPR, CCPA, etc.): Adherence to relevant data privacy regulations is crucial to avoid legal penalties and maintain public trust.
- Data Access Control and User Permissions: Implementing strict access control measures, including role-based access control, prevents unauthorized access to sensitive data.
Advanced Data Validation and Cleaning Techniques
Data cleaning and validation are essential to eliminate errors and ensure data integrity. Raw poll data often contains inconsistencies, outliers, and missing values. Addressing these issues is crucial for generating reliable results. A robust poll data system should incorporate automated checks and manual review processes.
Effective data cleaning techniques include:
- Outlier Detection: Identify and handle data points significantly different from the rest of the dataset. Outliers might be errors or legitimate extreme values; careful investigation is necessary.
- Duplicate Removal: Identify and remove duplicate entries to avoid overrepresentation of certain responses.
Further steps for ensuring data quality:
- Using Data Validation Rules to Ensure Data Integrity: Implement rules to check for inconsistencies and invalid entries during data entry.
- Implementing Automated Checks for Inconsistencies: Automated checks flag potential errors for review and correction.
- Handling Missing Data (Imputation Techniques): Employ appropriate imputation techniques to handle missing data, minimizing bias.
- The Role of Data Visualization in Identifying Errors: Visualizing data through charts and graphs can help identify patterns and anomalies that may indicate errors.
Robust Data Analysis and Reporting
Statistical methods are crucial for analyzing poll data and drawing meaningful conclusions. A robust poll data system should provide tools for conducting appropriate statistical analyses and generating clear, concise reports.
Key aspects of data analysis and reporting include:
- Appropriate Statistical Tests for Different Types of Data: Employ appropriate statistical tests based on the type of data (e.g., t-tests, ANOVA, chi-square tests).
- Confidence Intervals and Margin of Error Calculations: Calculate and report confidence intervals and margins of error to quantify the uncertainty associated with the results.
- Visualizing Data Effectively Using Charts and Graphs: Use clear and informative charts and graphs to present data effectively and highlight key findings.
- Generating Customized Reports Tailored to Specific Needs: The system should allow generating reports customized to different stakeholders' needs.
Conclusion
Building a robust poll data system involves a multifaceted approach encompassing accurate data collection, secure storage, thorough data validation, and robust data analysis and reporting. Accurate poll data is essential for informed decision-making in various fields, from market research to political analysis. By implementing the strategies discussed in this article, you can ensure your poll data provides valuable insights and supports informed decisions. Invest in a robust poll data system today!
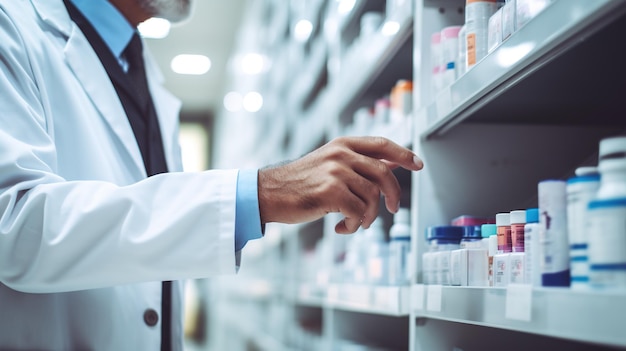