Exploring The Cognitive Limits Of Artificial Intelligence
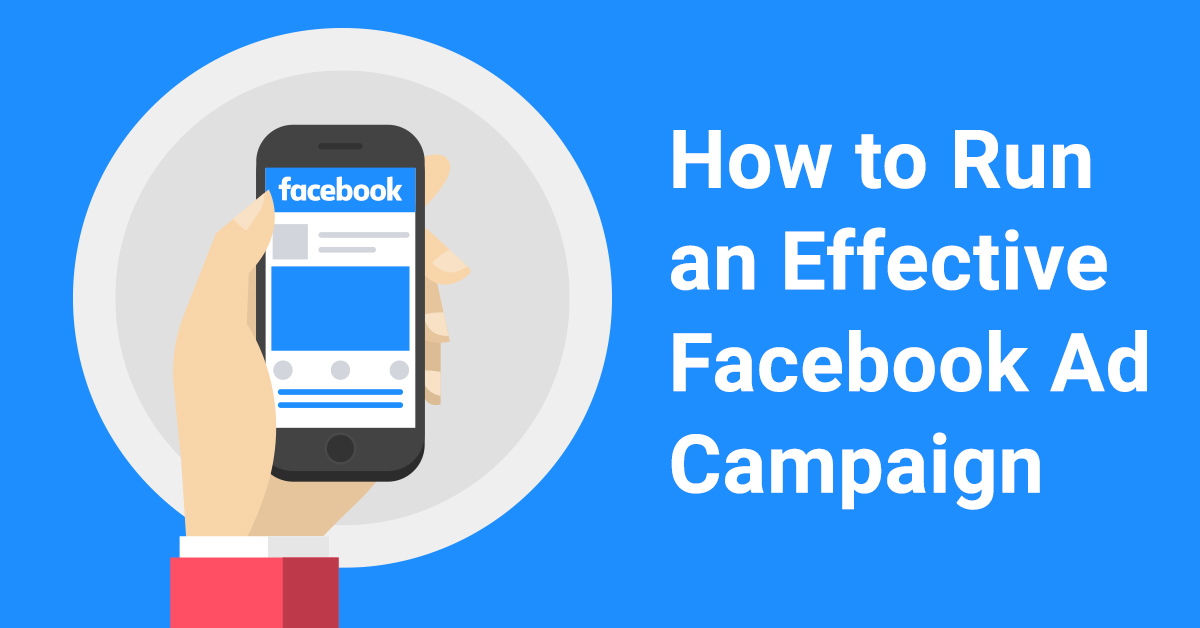
Table of Contents
The Lack of Common Sense Reasoning in AI
A fundamental difference between human and artificial intelligence lies in common sense reasoning. Humans effortlessly navigate everyday situations using implicit knowledge and contextual understanding, something that remains a significant hurdle for AI. While AI can excel at specific tasks, it often struggles with situations requiring even basic common sense. This gap highlights a crucial limitation in current AI systems.
- Examples of AI struggles: Imagine asking an AI to identify a picture of a cat sitting on a mat outdoors. While AI excels at image recognition, it may struggle to understand the implied relationship – that the cat is likely sitting on the mat, rather than in it or beside it. Another example is interpreting sarcasm or nuanced language.
- The role of implicit knowledge: Human reasoning relies heavily on implicit knowledge – information we understand intuitively without needing explicit explanation. This is difficult to program into AI systems. Contextual understanding is equally crucial, allowing us to interpret information based on its surrounding circumstances.
- Current research efforts: Researchers are exploring various avenues to bridge this gap. Knowledge graphs, which represent information as interconnected nodes, and neural-symbolic AI, which combines neural networks with symbolic reasoning, are promising approaches for imbuing AI with common sense reasoning capabilities.
The Problem of Generalization and Transfer Learning in AI
Generalization and transfer learning are critical aspects of intelligent behavior. Generalization refers to an AI's ability to apply knowledge learned from one set of data to new, unseen data. Transfer learning involves applying knowledge gained from solving one task to solve a different but related task. Current AI systems, particularly those based on deep learning, often struggle with both.
- Examples of AI generalization failures: An AI trained to identify cats in one set of images may fail to recognize cats in images with different lighting, angles, or breeds. Similarly, an AI trained to translate English to French might struggle with translating English legal documents into French due to the specific terminology.
- Limitations of current machine learning: Many machine learning models are data-hungry, requiring vast amounts of labeled data for training. This can lead to overfitting, where the model performs well on the training data but poorly on new data. The inherent limitations of current architectures also contribute to this problem.
- The role of data bias: Data bias is a significant obstacle to generalization. If the training data is biased, the resulting AI system will also be biased, hindering its ability to generalize accurately to diverse datasets.
The Absence of Consciousness and Self-Awareness in AI
The question of consciousness and self-awareness in AI is a complex philosophical debate. While AI can simulate human-like intelligence in specific domains, it remains far from possessing genuine consciousness or self-awareness.
- Arguments against conscious AI: Many argue that consciousness is an emergent property of complex biological systems and cannot be replicated in artificial systems. The subjective experience, or qualia, of consciousness is also a significant challenge to replicate.
- Defining and measuring consciousness: Measuring consciousness, even in humans, remains a significant scientific challenge. Defining and measuring consciousness in machines presents even greater difficulties.
- Ethical implications: The creation of conscious or self-aware AI raises profound ethical questions regarding rights, responsibilities, and potential risks.
The Limitations of Current AI Architectures
Current dominant AI architectures, like deep learning, exhibit both strengths and weaknesses. Deep learning excels at pattern recognition and data analysis but faces limitations when it comes to complex reasoning, common sense, and generalization.
- Limitations of deep learning: Deep learning models often struggle with tasks requiring symbolic reasoning, causal inference, or explainability. They can also be vulnerable to adversarial attacks, where subtle manipulations of input data can lead to incorrect predictions.
- Computational and data requirements: Training advanced AI systems demands enormous computational resources and vast quantities of data, raising concerns about energy consumption and environmental impact.
- Alternative architectures: Researchers are actively exploring alternative architectures and approaches, such as hybrid models combining deep learning with symbolic reasoning, neuromorphic computing, and biologically inspired AI, to address the limitations of current systems.
Navigating the Future of AI Cognition
In conclusion, current AI systems face significant cognitive limitations, including the lack of common sense reasoning, difficulties with generalization and transfer learning, the absence of consciousness, and the limitations of current architectures. Understanding these limitations is paramount for responsible AI development. While the field continues to advance at a rapid pace, it’s crucial to acknowledge these boundaries. The future of AI cognition will likely involve addressing these challenges through innovative architectures, improved learning algorithms, and a deeper understanding of the nature of intelligence itself. We encourage you to delve deeper into the fascinating and complex topic of the cognitive limits of artificial intelligence. Explore further research from leading AI institutions and publications to better understand the challenges and possibilities in this rapidly evolving field. Engage in discussions and contribute to the responsible development of AI, ensuring its benefits are maximized while mitigating potential risks associated with its limitations.
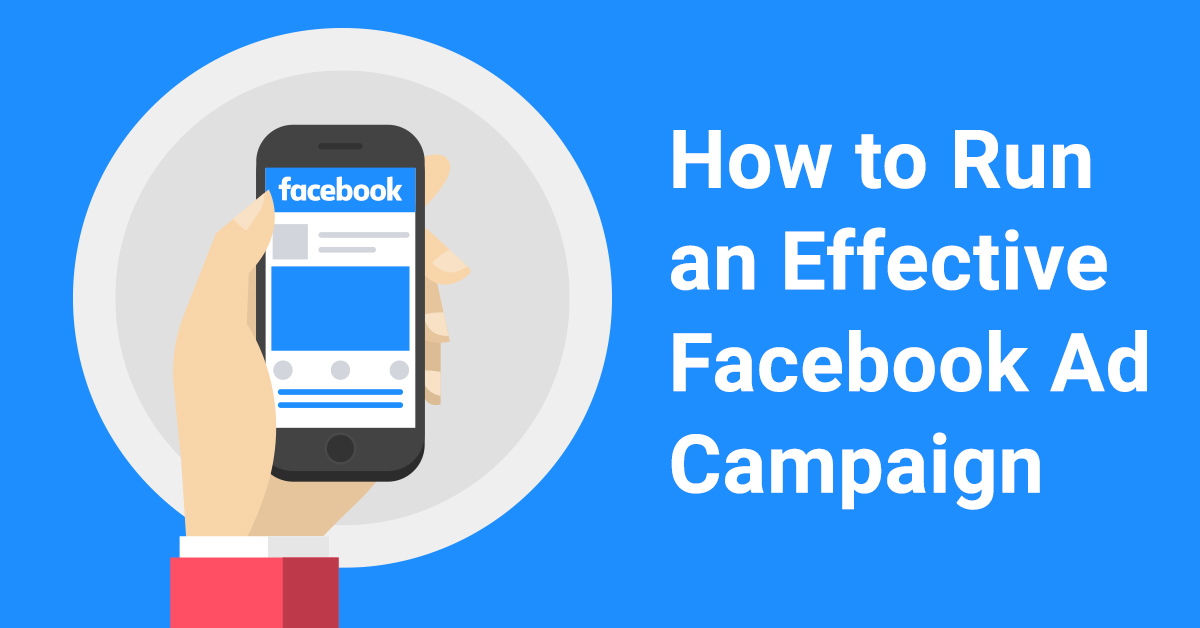
Featured Posts
-
Green Bay Packers Two Chances For A 2025 International Game
Apr 29, 2025 -
Chinas Huawei Develops Exclusive Ai Chip To Rival Nvidia
Apr 29, 2025 -
Antlaq Fealyat Fn Abwzby Fy 19 Nwfmbr Dlyl Shaml
Apr 29, 2025 -
Minnesota Immigrant Workforce Upward Mobility And Higher Paying Employment
Apr 29, 2025 -
Is A Tremors Series Coming To Netflix Everything We Know
Apr 29, 2025