How AI Thinks: A Surprisingly Simple Explanation
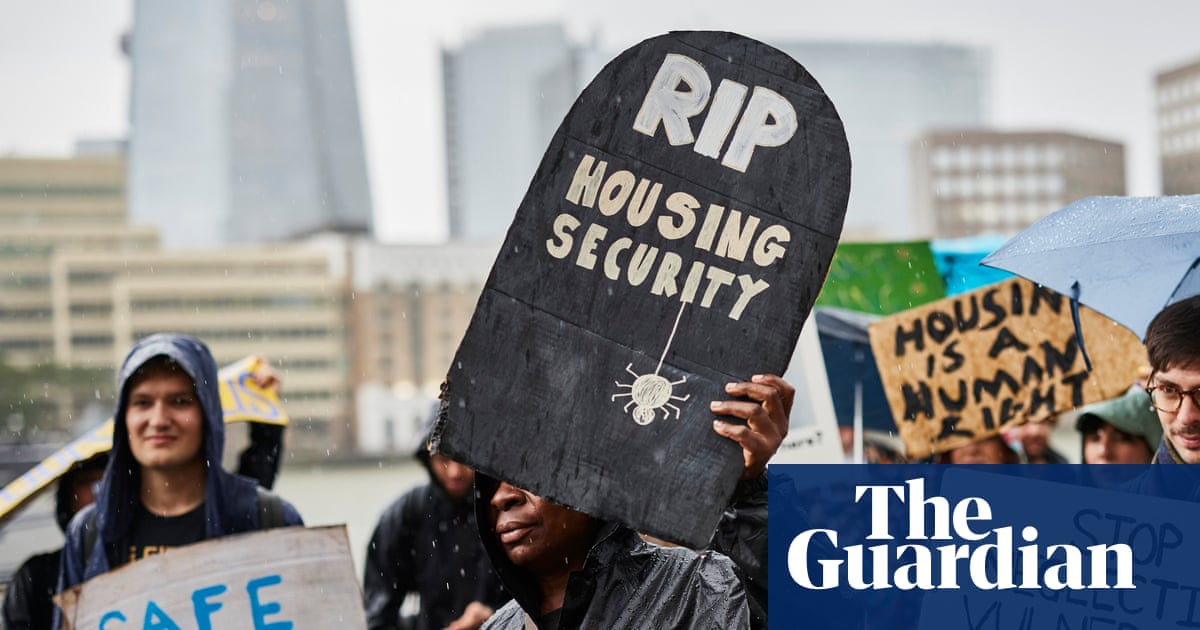
Table of Contents
The Core of AI Thinking: Data and Algorithms
At the heart of AI thinking lies a powerful combination: data and algorithms. AI "thinks" by processing vast amounts of data using sophisticated algorithms. These algorithms are essentially sets of instructions that tell the AI how to analyze the data and what to look for. The type of data plays a crucial role. We see two main types:
-
Structured Data: This is neatly organized data, easy for computers to understand, like data in a spreadsheet or database. Think of things like customer details, financial transactions, or sensor readings.
-
Unstructured Data: This is less organized data, like text, images, audio, and video. Processing this requires more advanced techniques.
The algorithms learn from this data through various methods:
-
Supervised Learning: This is like teaching a child. The algorithm is trained on a dataset where each data point is labeled with the correct answer. For example, in image recognition, you'd show the algorithm thousands of images of cats and dogs, each labeled accordingly. The algorithm learns to identify features that distinguish cats from dogs.
-
Unsupervised Learning: Here, the algorithm explores the data without pre-defined labels, identifying patterns and structures on its own. For example, analyzing customer purchase history to group customers into segments based on their buying behavior.
-
Reinforcement Learning: This is a trial-and-error approach where the algorithm learns through rewards and penalties. It takes actions, receives feedback, and adjusts its strategy to maximize rewards. A prime example is an AI playing a game like chess or Go. It learns by playing numerous games and receiving positive reinforcement (a win) or negative reinforcement (a loss). This shapes its strategy over time. The algorithm learns to make better decisions by receiving feedback.
Neural Networks: Mimicking the Brain
Artificial neural networks (ANNs) are the foundation of many advanced AI systems. Inspired by the structure and function of the human brain, these networks consist of interconnected nodes, or "neurons," organized in layers. Information flows between these nodes, and the connections between them have associated "weights" that determine the strength of the signal.
-
Network Layers: A typical neural network has an input layer (receiving the data), hidden layers (processing the data), and an output layer (producing the result).
-
Backpropagation: This is a crucial process where the network adjusts the weights of its connections based on errors in its predictions. If the network makes a mistake, backpropagation helps it refine its understanding and improve its accuracy over time.
-
Simple Analogy: Imagine a network of pipes with valves. The data flows through the pipes, and the valves (weights) control the flow. Backpropagation is like adjusting the valves to ensure the right amount of water flows to the correct destination.
Deep Learning: Taking it to the Next Level
Deep learning takes neural networks to the next level by using multiple layers, hence the term "deep." This architecture enables the processing of highly complex patterns and relationships in data. Two prominent types are:
-
Convolutional Neural Networks (CNNs): These excel at processing visual data, making them ideal for image recognition, object detection, and medical imaging.
-
Recurrent Neural Networks (RNNs): These are designed to handle sequential data like text and time series, useful for natural language processing, speech recognition, and machine translation.
Deep learning powers many real-world applications, including:
- Self-driving cars: Analyzing sensor data to navigate roads and avoid obstacles.
- Medical diagnosis: Identifying diseases from medical images with high accuracy.
- Fraud detection: Analyzing financial transactions to detect suspicious activity.
However, deep learning isn't without limitations. It often requires massive datasets and significant computational resources. Furthermore, the "black box" nature of deep learning models can make it difficult to understand how they arrive at their decisions.
Beyond Neural Networks: Other AI Approaches
While neural networks are dominant, other AI techniques exist, each with its strengths and applications:
-
Expert Systems: These rule-based systems use human-defined knowledge to make decisions. They're effective for specific, well-defined problems.
-
Decision Trees: These offer a visual representation of decision-making, making them easy to understand and interpret.
-
Support Vector Machines (SVMs): Powerful tools for classification and regression tasks, known for their robustness and efficiency.
-
Genetic Algorithms: Inspired by natural selection, these algorithms evolve solutions over time, useful for optimization problems.
Conclusion
In essence, AI "thinks" by processing data through algorithms, often leveraging the power of neural networks, particularly deep learning models, or other methods depending on the specific task. While the underlying processes are complex, the fundamental principles are surprisingly straightforward. The ability of AI to learn from data, refine its understanding, and make informed decisions relies on these fundamental building blocks.
Learn more about deep learning and its applications, or explore different types of AI algorithms to further deepen your understanding of this fascinating field. The seeming complexity of AI thinking masks a surprisingly simple core – a testament to the ingenuity of human design.
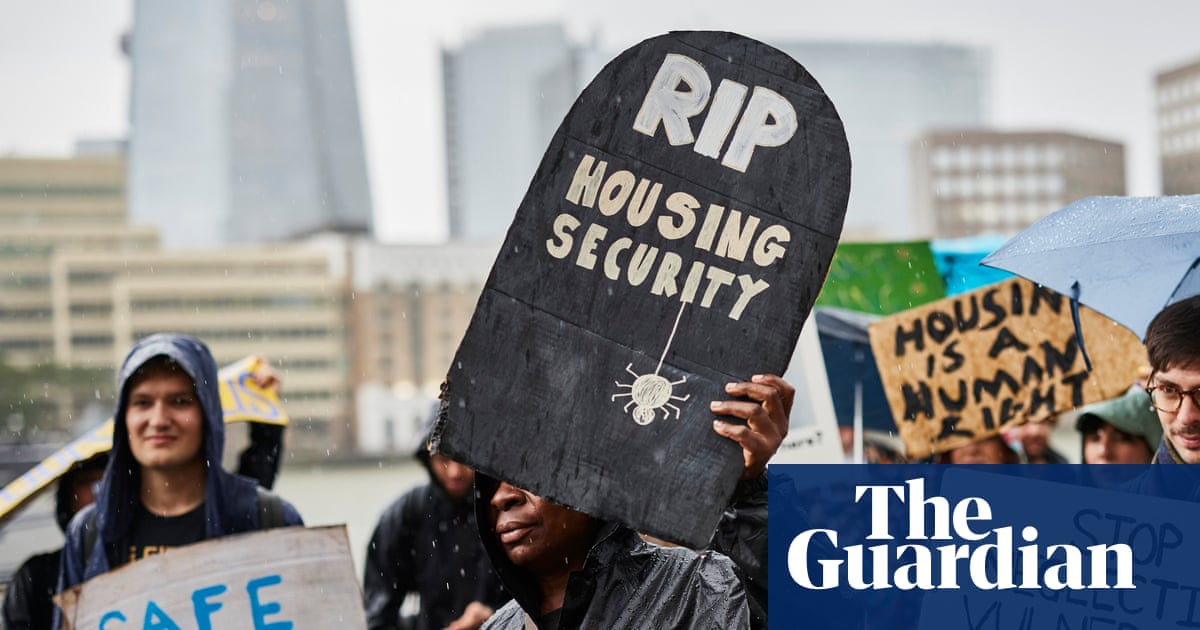
Featured Posts
-
Canada Election 2023 Mark Carneys Faltering Campaign Momentum
Apr 29, 2025 -
Us Research Exodus How Countries Are Competing For Top Talent After Funding Cuts
Apr 29, 2025 -
Hengrui Pharma Secures China Regulator Approval For Hong Kong Ipo
Apr 29, 2025 -
Georgia Law Enforcement Officer Killed Another Wounded In Traffic Stop
Apr 29, 2025 -
Harvard Faces Trump Administration In Court Over Funding Cuts
Apr 29, 2025
Latest Posts
-
Navigating The Difficulties Of All American Manufacturing
Apr 29, 2025 -
The Struggle To Make Products Entirely In America
Apr 29, 2025 -
Why All American Production Remains A Challenge
Apr 29, 2025 -
The High Cost Of All American Manufacturing
Apr 29, 2025 -
All American Products Why Domestic Manufacturing Is Tough
Apr 29, 2025