Improving The Accuracy Of Automated Visual Inspection Systems For Lyophilized Vials
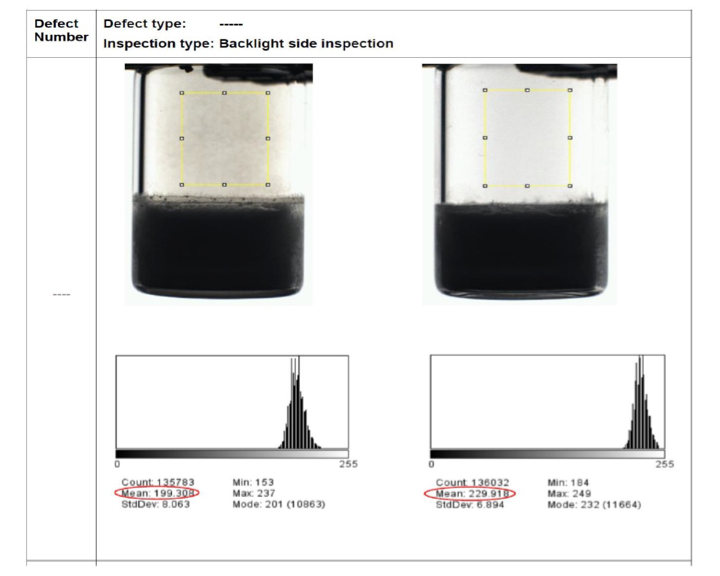
Table of Contents
Addressing Common Sources of Error in Automated Visual Inspection of Lyophilized Vials
The accuracy of automated visual inspection systems for lyophilized vials is susceptible to several factors. Understanding and mitigating these sources of error is paramount. Common challenges include:
- Inconsistent Lighting: Variations in lighting intensity and angle can cast shadows or create glare, leading to false positives (flagging acceptable vials as defective) or false negatives (missing actual defects). Uniform and optimized lighting is crucial for consistent image acquisition.
- Variations in Vial Fill Levels: Differences in the height of the lyophilized cake within vials can affect the detection of defects, particularly those located near the bottom or top of the cake. Consistent fill levels are essential, and the inspection system must be calibrated to account for acceptable variations.
- Subtle Defects: Detecting small cracks, particulate matter, or other subtle defects within the lyophilized cake presents a significant challenge. These defects are often difficult to discern even for trained human inspectors.
- Variations in Vial Color/Opacity: Differences in the color or opacity of vials, potentially due to variations in glass or manufacturing processes, can interfere with automated defect detection. The system must be calibrated to account for such variations.
!
!
Advanced Imaging Techniques for Enhanced Accuracy
Advanced imaging technologies are crucial in overcoming the limitations of traditional inspection methods. These techniques offer superior sensitivity and accuracy in defect detection:
- High-Resolution Cameras: Employing high-resolution cameras significantly increases the detail captured in each image, enabling the detection of minute cracks, particles, or other subtle imperfections that might be missed by lower-resolution systems.
- Multispectral Imaging: This technique uses multiple wavelengths of light to capture images, allowing for the differentiation between defects and benign variations in color or opacity. It provides a richer dataset for analysis, improving the accuracy of defect identification.
- Hyperspectral Imaging: Hyperspectral imaging captures images across a continuous range of wavelengths, providing even more detailed spectral information than multispectral imaging. This allows for the identification of defects based on their unique spectral signatures, leading to significantly improved accuracy.
- AI and Machine Learning: The integration of Artificial Intelligence (AI) and Machine Learning (ML) algorithms is transforming automated visual inspection. These algorithms can be trained on large datasets of images to learn to identify defects with high accuracy, even subtle ones difficult for human inspectors.
Successful implementations of these technologies can be found in various industries beyond pharmaceuticals, such as food inspection and semiconductor manufacturing, highlighting their versatility and effectiveness.
Optimizing Image Processing Algorithms and AI for Lyophilized Vial Inspection
Sophisticated image processing algorithms and AI play a pivotal role in optimizing the accuracy of automated visual inspection systems:
- Machine Learning Algorithms: Machine learning algorithms are trained on extensive datasets of images of both defective and non-defective vials. This training process allows the algorithm to learn to identify patterns and features characteristic of defects.
- Deep Learning: Deep learning, a subset of machine learning, can be particularly effective in identifying complex or subtle defects. Deep learning models can automatically extract relevant features from images without the need for manual feature engineering.
- Data Quality: The accuracy of AI models heavily relies on the quality and representativeness of the training dataset. A large, diverse dataset is crucial to ensure that the model can generalize well to new, unseen images.
- Quality Control Metrics: The performance of the image processing algorithms and AI models is assessed using various quality control metrics, including precision, recall, and the F1-score. These metrics provide a quantitative measure of the system’s accuracy.
Implementing Robust Quality Control Procedures for Automated Visual Inspection Systems
A comprehensive quality control program is essential for maintaining the accuracy and reliability of automated visual inspection systems over time:
- Regular Calibration and Maintenance: The inspection system needs regular calibration to ensure that the imaging components and algorithms are functioning correctly. Routine maintenance is also crucial for preventing malfunctions and ensuring optimal performance.
- Periodic Retraining of AI Models: AI models should be periodically retrained with updated datasets to account for changes in manufacturing processes, vial characteristics, or defect types.
- Statistical Process Control (SPC): Implementing SPC allows for continuous monitoring of the system's performance. This enables the identification of potential issues or drifts in accuracy before they impact product quality.
- Regular Audits and Validation: Regular audits and validation are essential to ensure that the automated inspection system complies with relevant regulatory guidelines, such as Good Manufacturing Practices (GMP).
Conclusion
Improving the accuracy of Automated Visual Inspection Systems for Lyophilized Vials requires a multifaceted approach. This involves employing advanced imaging techniques like high-resolution cameras and hyperspectral imaging, optimizing image processing algorithms and AI models through deep learning and robust training datasets, and establishing a stringent quality control program. By integrating these strategies, pharmaceutical companies can achieve significant reductions in labor costs, enhance product quality, increase throughput, and ensure regulatory compliance. Investing in advanced technologies such as high-resolution cameras and AI-powered image analysis software is a crucial step towards achieving these goals and ultimately protecting patient safety. Consider exploring these solutions and implementing best practices to optimize your own Automated Visual Inspection Systems for Lyophilized Vials and deliver superior pharmaceutical products.
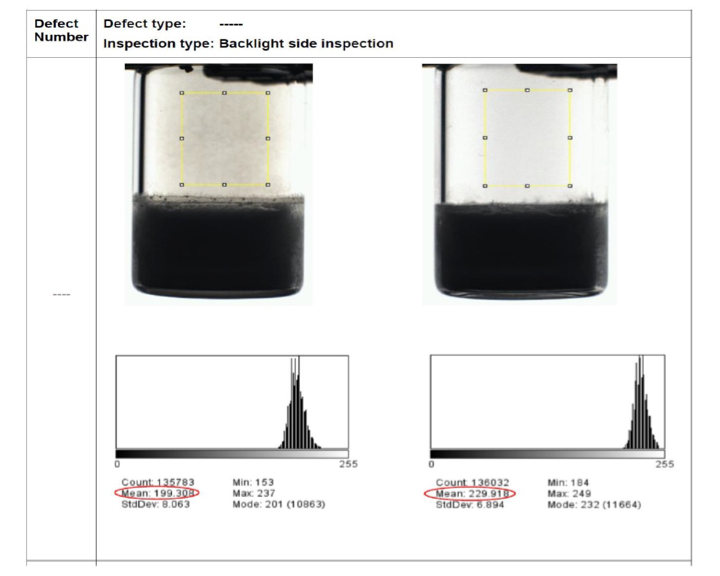
Featured Posts
-
Who Will Be The Next Pope Leading Contenders For The Papacy
May 12, 2025 -
Omagiu Adus Lui Thomas Mueller Momentele Memorabile De La Ultimul Sau Meci Cu Fanii Bayern
May 12, 2025 -
The Next Pope Key Factors Influencing The Papal Conclave
May 12, 2025 -
Nine Month Space Mission Details From Cbs News Report On Astronauts Stay
May 12, 2025 -
Close Contest Archbishop Bergan Edges Out Norfolk Catholic In District Final
May 12, 2025
Latest Posts
-
Stallone And Caines Unexpected On Screen Chemistry From Expendables To The Italian Job
May 12, 2025 -
Netherlands Plans Area Bans For Troublesome Asylum Seekers
May 12, 2025 -
Netherlands To Build Low Security Detention Centers For Asylum Seekers
May 12, 2025 -
Amsterdam Cafe Unveils Kings Day Mural A Tribute To Marjolein Fabers Ribbon Gate
May 12, 2025 -
The Impact Of Enhanced Border Security Fewer Arrests Higher Rejection Rates
May 12, 2025