Legacy Systems And AI: A Recipe For Disaster
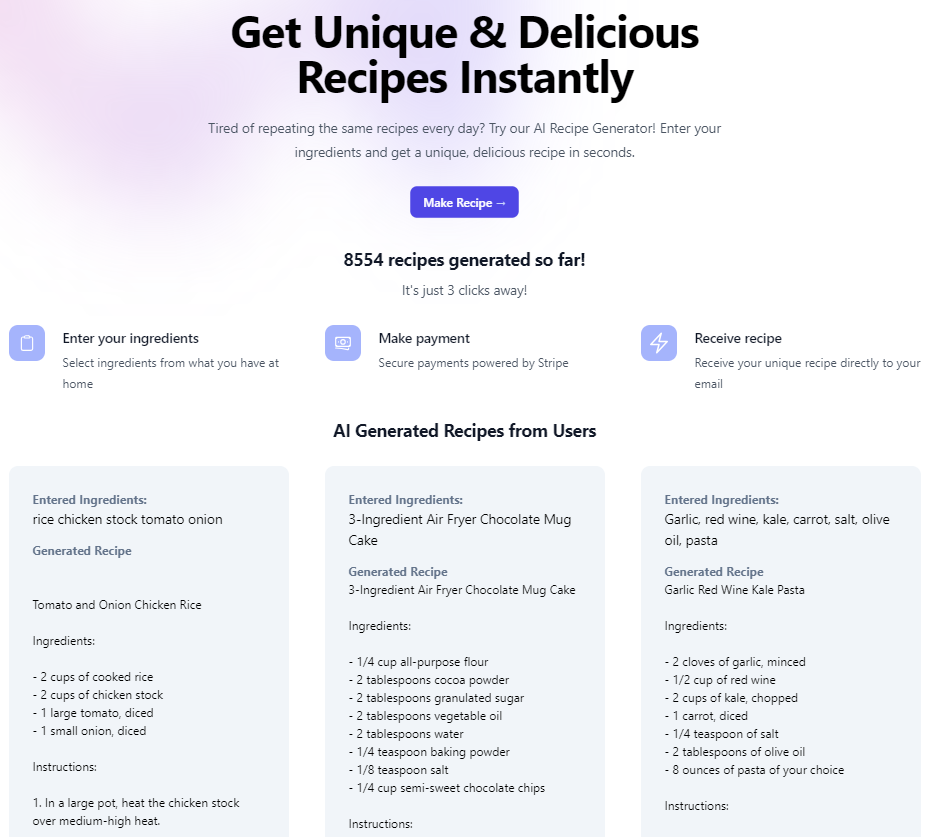
Table of Contents
Data Incompatibility and Migration Challenges
Integrating AI successfully requires clean, consistent, and readily accessible data. Legacy systems, however, often present significant hurdles in this area. The challenges of data incompatibility and migration are substantial obstacles in the path of a smooth AI integration.
Data Format Inconsistencies
Legacy systems frequently employ outdated data formats like flat files or proprietary databases, incompatible with modern AI algorithms that thrive on structured, easily processed data like JSON or CSV. This necessitates extensive data cleaning, transformation, and migration—a complex and time-consuming process prone to errors.
- Difficulties converting legacy data: Transforming data from legacy formats (e.g., converting a decades-old database into a usable CSV) can be incredibly challenging and resource-intensive.
- Risk of data loss during migration: The sheer volume of data and the complexity of the transformation process inherently increases the risk of data loss or corruption during migration. Robust backup and recovery strategies are critical.
- Need for robust data validation and quality checks: Ensuring data accuracy and consistency after migration is paramount. Rigorous validation and quality checks are essential to prevent flawed AI models.
- Cost and time associated with data transformation: The cost of hiring data scientists, engineers, and employing specialized tools for data transformation can be substantial, leading to project delays and budget overruns.
Data Silos and Integration Issues
Data is often scattered across various legacy systems, creating significant data silos. This fragmentation hinders AI's ability to access a comprehensive view of the data, limiting its ability to build accurate and effective models. Integrating these disparate data sources requires significant effort and can introduce inconsistencies and inaccuracies.
- Challenges in connecting different legacy systems: Legacy systems may use different protocols and technologies, making it difficult to establish seamless data flow between them.
- Issues with data governance and consistency across systems: Inconsistent data definitions and formats across different legacy systems introduce inaccuracies and inconsistencies. Establishing robust data governance is critical.
- Need for ETL (Extract, Transform, Load) processes: ETL processes are essential to extract data from various sources, transform it into a compatible format, and load it into a central data warehouse for AI processing. This is complex and time-consuming.
- Risk of incomplete or inaccurate AI models due to data silos: AI models trained on incomplete or inconsistent data from disparate silos will likely produce inaccurate and unreliable results, rendering the AI initiative ineffective.
Security Risks and Vulnerabilities
Integrating AI with legacy systems introduces significant security risks. Outdated security protocols in legacy systems create vulnerabilities that can be exploited by malicious actors, jeopardizing sensitive data and compromising the entire system.
Outdated Security Protocols
Legacy systems often lack modern security protocols such as robust encryption, multi-factor authentication, and regular security patching. This makes them easy targets for cyberattacks. Integrating AI with these systems exacerbates these risks.
- Exposure to data breaches due to insufficient security measures: Weak security in legacy systems can expose sensitive data used by AI algorithms, leading to significant data breaches.
- Difficulties implementing security patches on outdated systems: Outdated systems often lack the capability to receive and apply modern security patches, leaving them vulnerable to known exploits.
- Risk of malware infection affecting both legacy systems and AI components: A security breach in a legacy system can easily spread to the AI components, compromising the entire system.
- Need for enhanced security audits and penetration testing: Thorough security audits and penetration testing are crucial to identify and mitigate security vulnerabilities before integrating AI.
Lack of Scalability and Maintainability
Legacy systems are often not designed to handle the scale and complexity of AI applications. This can lead to performance bottlenecks and difficulties in maintaining the integrated system, creating further problems for AI initiatives.
- Limited processing power to handle AI workloads: Legacy systems may lack the processing power and memory to handle the computationally intensive tasks required by AI algorithms.
- Difficulties in scaling the system to accommodate increased data volume and processing demands: As AI applications grow, they require more resources. Legacy systems often struggle to scale efficiently.
- High maintenance costs associated with outdated infrastructure: Maintaining outdated hardware and software is expensive and time-consuming.
- Risk of system downtime and service disruptions: Outdated systems are more prone to failures and downtime, disrupting AI operations.
Cost and Time Overruns
Integrating AI with legacy systems is often fraught with unexpected challenges, leading to significant cost and time overruns. These unforeseen issues can derail even the most well-intentioned AI projects.
Unexpected Integration Challenges
The process of integrating AI with legacy systems often reveals unforeseen complexities and compatibility issues, resulting in delays and increased costs.
- Unexpected delays in data migration and system integration: The process is often more complex than anticipated, leading to unforeseen delays.
- Increased development costs due to complex workarounds and custom solutions: Developers may need to create custom solutions to bridge compatibility gaps between legacy systems and AI components, significantly increasing development costs.
- Need for specialized expertise in both legacy systems and AI: Finding professionals with expertise in both legacy systems and AI is challenging and expensive.
- Project scope creep and budget overruns: Unforeseen complexities can lead to scope creep and significant budget overruns.
The Hidden Costs of Maintaining Outdated Systems
The ongoing costs of maintaining legacy systems can quickly outweigh the benefits, especially when coupled with the expenses of AI integration.
- High operational costs associated with running outdated hardware and software: Running and maintaining legacy systems is often more expensive than newer, more efficient systems.
- Increased maintenance and support costs: Finding support for outdated systems can be challenging and expensive.
- Limited access to skilled personnel familiar with legacy systems: Finding personnel with expertise in legacy systems is becoming increasingly difficult.
- Risk of vendor lock-in and limited flexibility: Legacy systems can lead to vendor lock-in, limiting flexibility and increasing costs.
Conclusion
Integrating AI with legacy systems presents significant challenges, including data incompatibility, security vulnerabilities, and cost overruns. While the benefits of AI are undeniable, a thoughtful approach to legacy system modernization or a strategic migration plan is essential. Ignoring these risks can lead to a costly and inefficient implementation, potentially jeopardizing the entire AI initiative. Before embarking on AI integration, carefully assess the risks associated with your legacy systems and consider whether modernization or a phased approach offers a more sustainable and successful path to leveraging the power of AI. Don't let your legacy systems become a recipe for disaster; plan carefully for successful AI integration, considering legacy system modernization as a crucial step in your digital transformation journey.
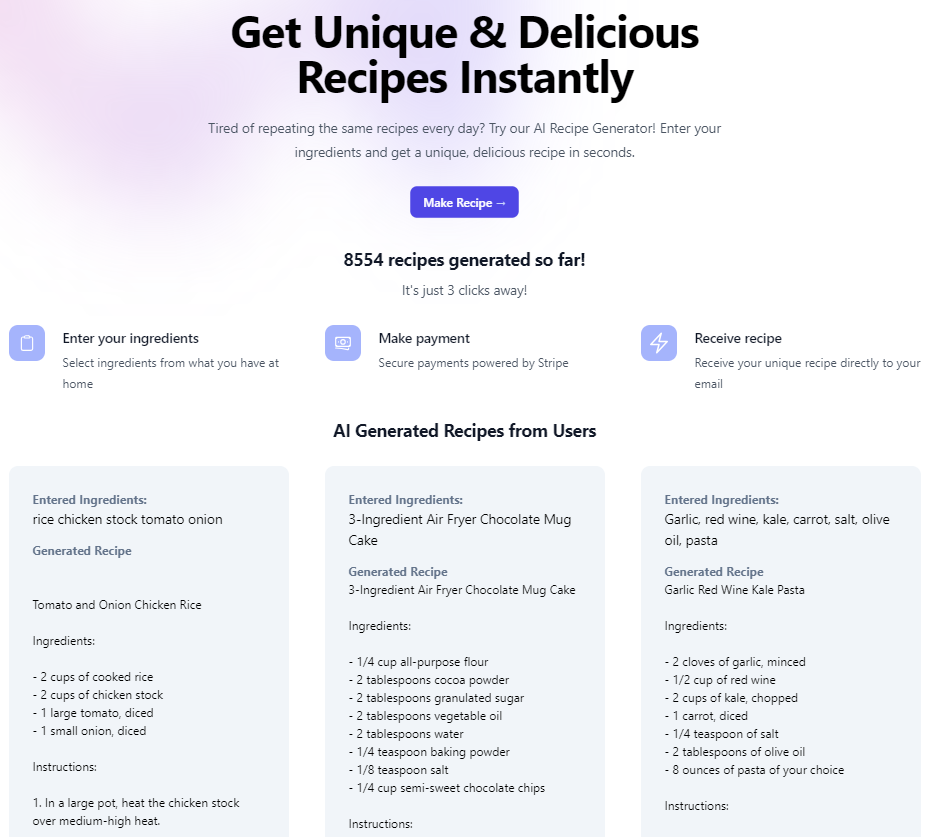
Featured Posts
-
Ripple Xrp Fourth Largest Crypto A Detailed Investment Analysis
May 01, 2025 -
Jokic I Jovic Na Evrobasketu Sedlacek Ocekuje Pojacanja
May 01, 2025 -
Prince William A Pensive Portrait Released By Kensington Palace
May 01, 2025 -
Auto Opladen In Noord Nederland Bespaar Geld Met Enexis Buiten Piekuren
May 01, 2025 -
Exploring The Nutritional Powerhouse Asparagus And Its Health Advantages
May 01, 2025