New CNIL Guidelines On AI: A Practical Guide For Compliance
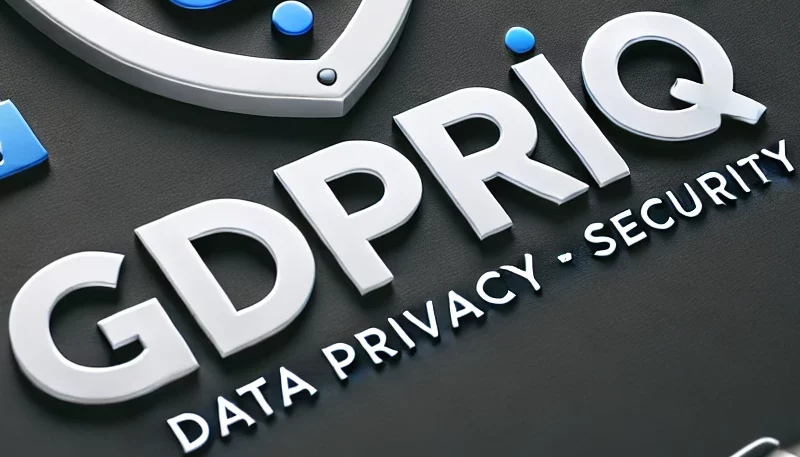
Table of Contents
Key Principles of the New CNIL Guidelines on AI
The CNIL's updated guidelines emphasize several core principles for responsible AI development and deployment, aligning closely with the broader principles of the GDPR. These principles are not merely suggestions; they represent legally binding requirements for organizations operating within France.
Data Minimization and Purpose Limitation
The principle of data minimization dictates that only the necessary personal data should be collected for specified, explicit, and legitimate purposes. This is particularly crucial in AI, where vast datasets are often used for training. Failing to adhere to this principle can lead to significant legal risks.
- Conducting Data Protection Impact Assessments (DPIAs): For high-risk AI systems (those likely to cause significant harm if improperly used), a DPIA is mandatory. This involves identifying potential risks, implementing mitigating measures, and documenting the entire process.
- Minimizing Data Collection in AI Development: Consider using synthetic data or federated learning techniques where possible to reduce reliance on real personal data. Anonymisation and pseudonymisation techniques should be explored and implemented whenever feasible.
- Purpose Limitation and AI Model Retraining: The initial purpose for which data was collected must be respected. Repurposing data for a different AI model requires obtaining fresh consent from data subjects or finding another justifiable legal basis.
Transparency and Explainability
Transparency and explainability are paramount. Users should understand how AI systems process their personal data and the logic behind decisions that affect them. While complete transparency might not always be feasible due to trade secrets, striving for explainable AI (XAI) is vital.
- Meaningful Information for Users: Provide clear and accessible information about how AI systems use their data, including the purpose, logic, and potential consequences. This might involve simplified explanations of algorithmic processes or providing examples of decision-making.
- Techniques for More Transparent Algorithms: Employ methods like feature importance analysis or decision tree visualization to make algorithmic processes more understandable. Consider using model cards to document AI model characteristics and limitations.
- Balancing Transparency and Trade Secrets: Organizations must find a balance between transparency requirements and protecting commercially sensitive information. This might involve providing summaries of processes rather than full algorithmic details.
Human Oversight and Accountability
Maintaining human control and establishing accountability mechanisms are non-negotiable. AI systems should not operate autonomously without human oversight, especially in high-risk contexts.
- Implementing Effective Human Oversight: Establish processes for monitoring AI system performance, identifying potential biases, and intervening when necessary. Regular audits and human-in-the-loop systems are crucial.
- Documenting AI System Decisions: Maintain detailed records of AI system decisions, including the data used, the algorithms applied, and the resulting outcomes. This is vital for accountability and potential redress.
- Addressing Errors and Biases: Develop mechanisms for detecting and correcting errors and biases in AI systems. Regular testing and validation, along with ongoing monitoring, are critical.
Specific Requirements for Different Types of AI Systems
The CNIL guidelines differentiate between high-risk and non-high-risk AI systems, imposing stricter requirements on the former.
High-Risk AI Systems
High-risk AI systems, such as those used in hiring, credit scoring, or law enforcement, are subject to more stringent compliance requirements. These systems necessitate rigorous testing and validation procedures to ensure fairness and accuracy.
- Specific Compliance Requirements: These include detailed DPIAs, robust risk mitigation strategies, and adherence to strict accuracy and fairness standards. Independent audits and conformity assessments may be required.
- Rigorous Testing and Validation: Thorough testing is crucial to identify and mitigate potential biases and errors before deployment. This involves evaluating the system’s performance against various metrics and diverse datasets.
- Conformity Assessment Process: The conformity assessment process might involve third-party audits to verify compliance with the CNIL's guidelines.
Non-High-Risk AI Systems
Even AI systems not deemed high-risk must still adhere to core data protection principles. While the level of scrutiny is lower, neglecting data protection can still lead to legal repercussions.
- CNIL Recommendations for Low-Risk AI: The CNIL provides recommendations for data privacy management in these applications, focusing on responsible data handling and security.
- Continued Importance of Data Protection Principles: Even in low-risk scenarios, the principles of data minimization, purpose limitation, and transparency remain critical.
- Best Practices for Data Security and Management: Implement robust data security measures, including encryption and access controls, to protect personal data used in AI systems.
Practical Steps for Achieving Compliance with the New CNIL Guidelines on AI
Achieving compliance requires a proactive approach involving audits and implementation of necessary changes.
Conducting a GDPR and CNIL AI Compliance Audit
A thorough audit is the first step in assessing compliance. This involves reviewing existing AI systems and processes against the new CNIL guidelines and GDPR requirements.
- Step-by-Step Audit Approach: Systematically assess each AI system, examining data collection practices, algorithmic processes, and oversight mechanisms.
- Key Areas to Focus On: Pay close attention to data minimization, transparency, accountability, and risk mitigation strategies.
- Tools and Resources: Utilize compliance checklists, data mapping tools, and risk assessment frameworks to aid in the audit process.
Implementing Necessary Changes and Documentation
Once the audit is complete, implement necessary changes to bring systems into compliance and maintain meticulous documentation throughout the process.
- Steps to Implement Changes: This might involve modifying data collection practices, enhancing transparency measures, or strengthening human oversight.
- Robust Documentation: Maintain comprehensive documentation of all processes, decisions, and changes made to achieve compliance. This is crucial for demonstrating accountability.
- Ongoing Compliance Monitoring: Establish a process for ongoing monitoring to ensure continued compliance with the evolving regulatory landscape.
Conclusion
Successfully navigating the complexities of the new CNIL guidelines on AI requires a proactive and comprehensive approach. By understanding the key principles of data minimization, transparency, and accountability, and by taking the necessary steps to conduct a thorough compliance audit and implement necessary changes, organizations can ensure they are meeting their legal obligations and maintaining public trust. Don't wait until it's too late – start your journey towards CNIL AI compliance today. Learn more about the specific requirements and best practices by downloading our comprehensive guide to CNIL guidelines on AI.
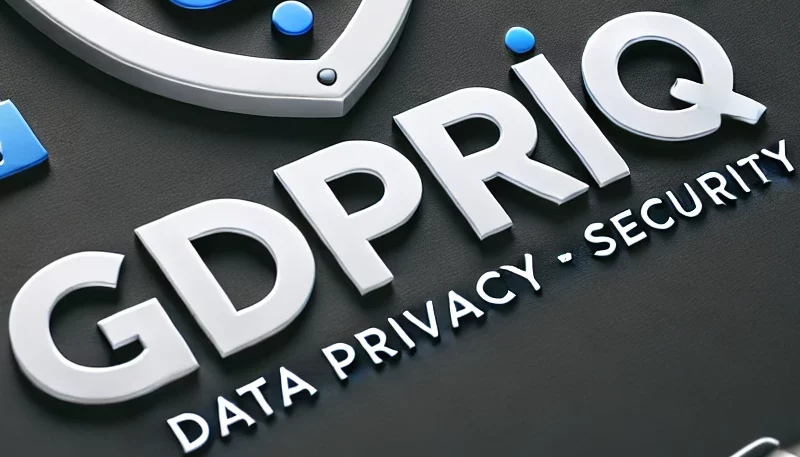
Featured Posts
-
Mo U Signed Schneider Electric Vignan University Center Of Excellence Opens In Vijayawada
Apr 30, 2025 -
Unos Studentky Sone Rozhodovanie Sudu O Obnove Konania V Stredu
Apr 30, 2025 -
Trade Shows Key Marketing Touchpoints For Schneider Electrics Success
Apr 30, 2025 -
Watch Untucked Ru Pauls Drag Race Season 16 Episode 11 Online Free Streaming Guide
Apr 30, 2025 -
New Cnil Guidelines On Ai A Practical Guide For Compliance
Apr 30, 2025
Latest Posts
-
Channing Tatum Moves On Romance With Inka Williams Following Zoe Kravitz Split
Apr 30, 2025 -
Is Channing Tatum Dating Inka Williams Relationship Speculation Mounts
Apr 30, 2025 -
Unconfirmed Zoe Kravitz And Noah Centineos Potential Romance
Apr 30, 2025 -
The Truth Behind Zoe Kravitz And Noah Centineos Dating Rumors
Apr 30, 2025 -
Channing Tatum Dating Australian Model Inka Williams Following Divorce
Apr 30, 2025