Outdated Business Apps: Obstructing Your AI Strategy
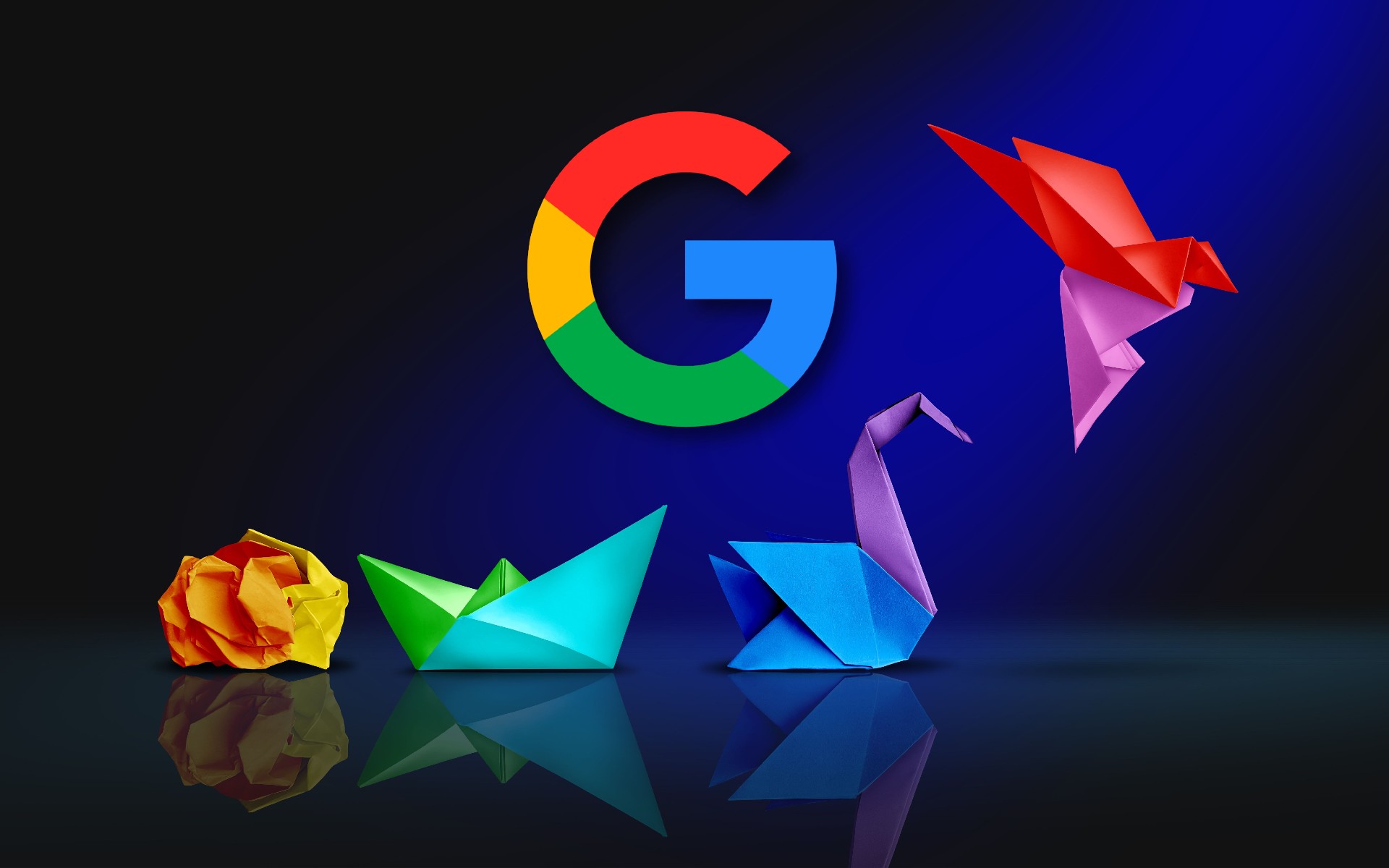
Table of Contents
Data Silos and Integration Challenges
Outdated apps frequently present significant hurdles to effective AI implementation due to inherent data integration problems. The fragmented nature of data stored across various systems creates bottlenecks that hinder the AI process.
The Problem of Inconsistent Data
Outdated apps often store data in disparate formats and locations, creating significant data silos. This fragmentation makes it incredibly difficult, if not impossible, to consolidate data for AI training and analysis. A robust AI strategy requires a unified view of your data, something legacy systems rarely provide.
- Lack of standardized data formats: Different applications may use different data formats (e.g., CSV, XML, proprietary formats), making it challenging to combine and analyze data effectively.
- Difficulties in data extraction: Extracting data from outdated apps can be complex and time-consuming, requiring specialized skills and tools.
- Increased risk of data errors: Manual data extraction and transformation processes increase the risk of human error, leading to inaccurate data and compromised AI model accuracy.
- Inconsistent data quality impacting AI model accuracy: Inconsistent data quality across different sources directly impacts the accuracy and reliability of AI models, rendering them less effective.
The Cost of Manual Data Integration
Manually integrating data from multiple outdated systems is a significant drain on resources. It's time-consuming, expensive, and error-prone, delaying AI implementation and increasing overall project costs.
- High labor costs: Significant human resources are required to manually extract, clean, transform, and load data from various sources.
- Increased risk of errors: Manual processes are inherently prone to human error, leading to inaccuracies and inconsistencies in the integrated data.
- Bottleneck in data flow: Manual data integration creates a significant bottleneck, slowing down the entire AI development and deployment process.
- Slower AI project timelines: The delays caused by manual data integration significantly extend AI project timelines, impacting time-to-market and ROI.
Scalability and Performance Limitations
The limitations of outdated business applications extend beyond data integration, significantly impacting the scalability and performance necessary for effective AI.
Inefficient Processing and Storage
Outdated apps often struggle with the demands of large datasets required for effective AI. This results in performance bottlenecks, slow processing times, and an inability to scale with growing data volumes.
- Limited processing power: Older systems may lack the processing power needed to handle the computational demands of AI algorithms.
- Inadequate storage capacity: Outdated systems often lack the storage capacity to accommodate large datasets required for AI training and model building.
- Slow response times: Slow processing and retrieval of data lead to slow response times, hindering the effectiveness of AI applications.
- Inability to scale with growing data volumes: As data volumes increase, outdated systems struggle to keep up, limiting the potential of AI initiatives.
Lack of API Integration
Modern AI solutions rely heavily on APIs (Application Programming Interfaces) for seamless integration. Outdated apps often lack robust API support, creating significant hurdles to interoperability with AI platforms.
- Difficulty in connecting to AI platforms: Lack of APIs makes it difficult to connect outdated apps to modern AI platforms, preventing data exchange.
- Limited data exchange capabilities: Without APIs, data exchange between legacy systems and AI platforms becomes cumbersome and inefficient.
- Increased development complexity for integrations: Custom integrations become necessary, significantly increasing development time and cost.
Security Risks and Compliance Issues
Outdated business apps pose significant security and compliance risks that can directly impact your AI strategy and potentially lead to serious consequences.
Vulnerability to Cyber Threats
Outdated apps often lack the latest security patches and updates, making them vulnerable to cyberattacks and data breaches. This poses a significant risk to sensitive data used in AI initiatives.
- Increased risk of data breaches: Vulnerable systems are easier targets for cyberattacks, leading to potential data breaches and loss of sensitive information.
- Compliance violations: Security breaches can lead to violations of data privacy regulations, resulting in significant penalties.
- Reputational damage: Data breaches can severely damage a company's reputation, leading to loss of customer trust and business.
- Financial losses: The costs associated with data breaches, including fines, legal fees, and remediation efforts, can be substantial.
Difficulty in Meeting Compliance Standards
Outdated apps may not comply with relevant data privacy regulations (like GDPR, CCPA), potentially hindering AI projects that involve sensitive personal data.
- Non-compliance with regulations: Failure to comply with data privacy regulations can result in significant fines and legal repercussions.
- Potential for hefty fines: Regulatory bodies impose substantial fines for non-compliance with data privacy laws.
- Legal ramifications: Non-compliance can lead to lawsuits and other legal actions.
Strategies for Overcoming Obstacles
Addressing the challenges posed by outdated business apps requires a strategic approach focusing on modernization and improved data integration.
App Modernization and Cloud Migration
Modernizing existing applications or migrating them to the cloud offers a powerful solution to many of the issues associated with outdated apps, creating a robust foundation for successful AI implementation.
- Improved data integration: Modern applications and cloud platforms offer better data integration capabilities, facilitating seamless data flow for AI processing.
- Enhanced scalability: Cloud-based solutions provide scalability and flexibility to handle growing data volumes and computational demands.
- Increased security: Cloud providers offer robust security features and measures to protect data from cyber threats.
- Reduced maintenance costs: Cloud-based solutions often reduce the need for on-premise infrastructure and IT support, resulting in lower maintenance costs.
Investing in Data Integration Tools
Utilizing robust data integration platforms can help consolidate data from various sources, including outdated apps, for efficient AI processing.
- Automated data integration: Data integration tools automate the process of extracting, transforming, and loading data from different sources.
- Improved data quality: Data integration tools can help clean and standardize data, ensuring higher data quality for AI models.
- Reduced manual effort: Automation significantly reduces manual effort, freeing up valuable resources.
- Faster AI project deployment: Improved data integration accelerates the AI project deployment process, enabling faster time-to-market.
Conclusion
Outdated business applications represent a significant hurdle to the successful implementation of an effective AI strategy. The challenges related to data integration, scalability, security, and compliance cannot be ignored. By addressing these issues through app modernization, cloud migration, and strategic investment in data integration tools, businesses can pave the way for seamless AI integration and unlock the true potential of AI-driven innovation. Don't let outdated business apps obstruct your AI strategy – take action today to modernize your infrastructure and unlock the power of AI.
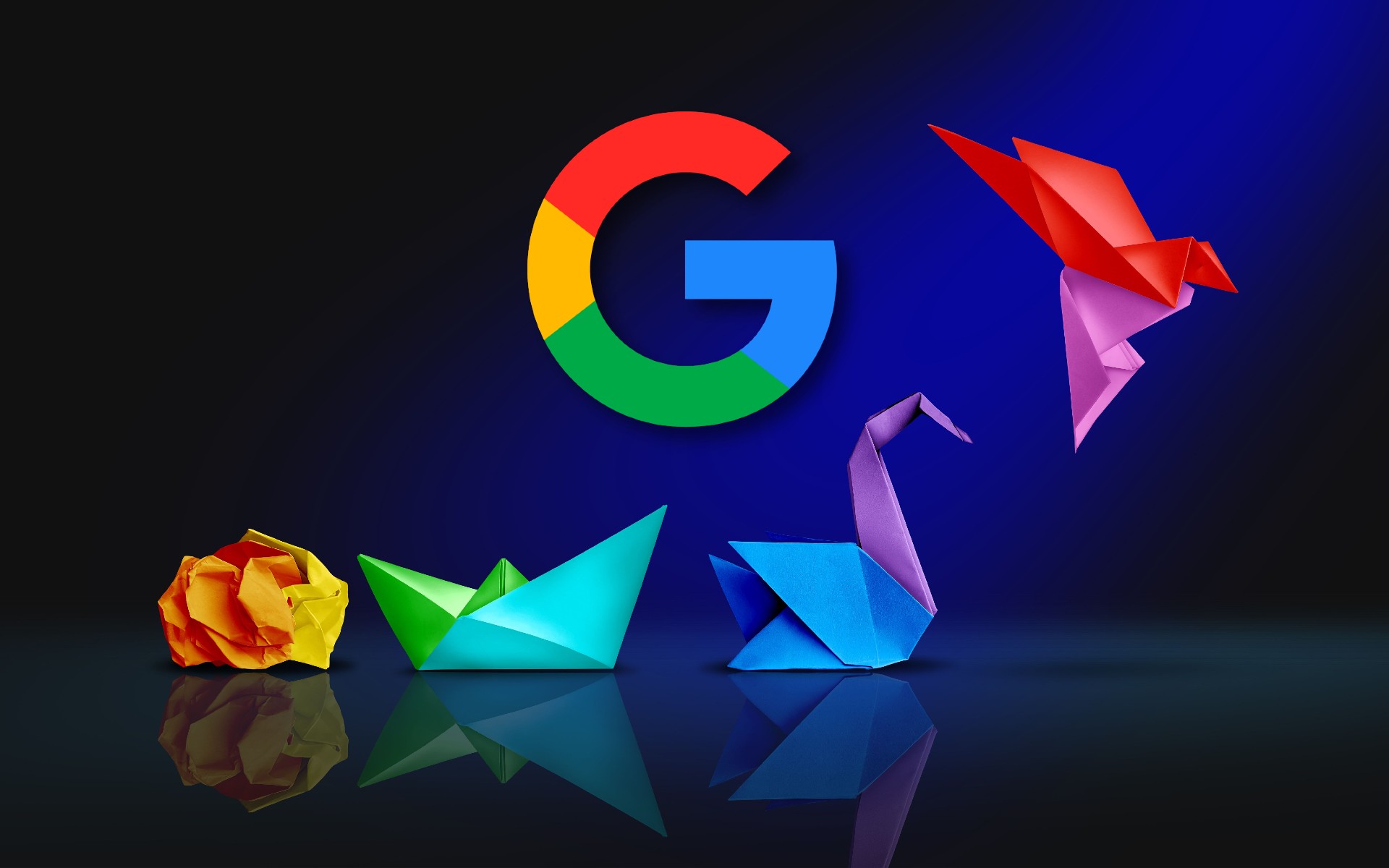
Featured Posts
-
Lempron Tzeims Analyontas Tin Istoriki Epidosi Ton 50 000 Ponton
May 01, 2025 -
Priscilla Pointer 1923 2023 Remembering A Legacy In Acting
May 01, 2025 -
Boxing Seminar With Ace Power Promotion Learn The Skills On March 26th
May 01, 2025 -
Farmers And Foragers Charlotte Barn Available For Purchase
May 01, 2025 -
Join The Arc Raiders Second Public Test Gameplay Improvements
May 01, 2025
Latest Posts
-
Aj Kshmyr Ykjhty Ywm Pwry Qwm Ka Sath
May 02, 2025 -
Kshmyr Ywm Ykjhty Mzahre Awr Tqrybat Ka Jayzh
May 02, 2025 -
Pakstan Myn Kshmyr Ykjhty Ywm Ke Shandar Azhar
May 02, 2025 -
Kshmyr Bhart Ke Lye Mdhakrat Ka Amkan Awr Fwjy Apshnz
May 02, 2025 -
Xrp Cryptocurrency A Beginners Guide
May 02, 2025