Revealing The Mechanisms Of AI "Thought"
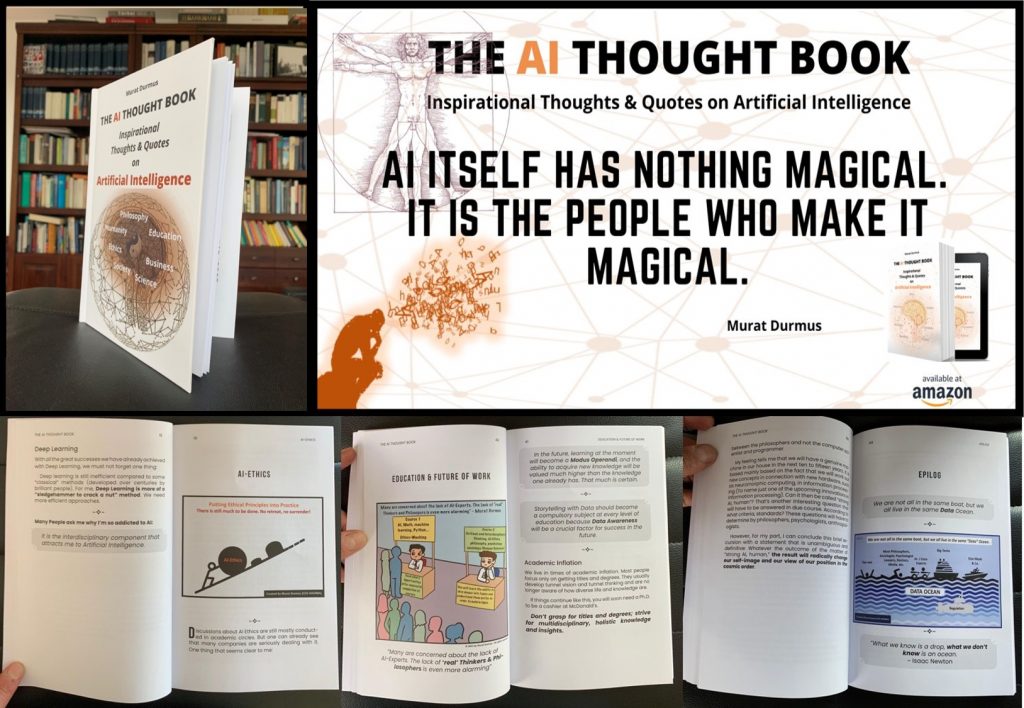
Table of Contents
Neural Networks: The Foundation of AI Thinking
At the heart of most advanced AI systems lie neural networks, complex structures inspired by the human brain. These networks consist of interconnected nodes, organized in layers, that process information. Think of it as a vast, interconnected web where each node receives input, performs a calculation, and passes the result to other nodes. This layered architecture allows the network to learn and extract patterns from data, mimicking—in a simplified way—the brain's ability to learn and make decisions.
Types of Neural Networks
Several types of neural networks cater to different tasks. Their structure and function vary, allowing them to excel in specific applications:
- Feedforward Neural Networks: The simplest type, where information flows in one direction, from input to output. Often used for basic classification tasks.
- Convolutional Neural Networks (CNNs): Specialized for image recognition and processing. They use convolutional layers to detect features in images, making them highly effective in applications like object detection and facial recognition.
- Recurrent Neural Networks (RNNs): Designed for sequential data, like text and time series. Their ability to retain information from previous inputs makes them ideal for natural language processing (NLP) tasks, machine translation, and speech recognition. RNN variations like LSTMs and GRUs address the vanishing gradient problem, improving performance on longer sequences.
Learning Through Data
Neural networks learn through exposure to vast amounts of data. This process, often called training, involves adjusting the connections between nodes to minimize errors in the network's predictions. Three main learning paradigms exist:
- Supervised Learning: The network is trained on labeled data, where inputs are paired with the correct outputs. For example, an image recognition model might be trained on thousands of labeled images of cats and dogs.
- Unsupervised Learning: The network learns patterns from unlabeled data, identifying inherent structures and relationships without explicit guidance. Clustering algorithms are a prime example.
- Reinforcement Learning: The network learns through trial and error, receiving rewards for correct actions and penalties for incorrect ones. This is commonly used in robotics and game playing AI.
Challenges like data bias (where the training data reflects existing societal biases) and overfitting (where the model performs well on training data but poorly on new, unseen data) are significant hurdles in developing robust AI systems.
Algorithms and Decision-Making Processes
AI's ability to make decisions stems from sophisticated algorithms that process information and generate outputs. Understanding these processes reveals the core of AI "thought."
From Input to Output
The journey of information through an AI system typically involves these steps:
- Data Input: Raw data (images, text, sensor readings) is fed into the system.
- Preprocessing: Data is cleaned, transformed, and prepared for processing.
- Feature Extraction: Relevant features are extracted from the data.
- Algorithm Application: Algorithms analyze the extracted features.
- Output Generation: The AI system produces a result (classification, prediction, action).
Decision Trees and Other Algorithms
Various algorithms contribute to AI's decision-making prowess:
- Decision Trees: These algorithms create a tree-like structure to classify data based on a series of decisions. Easy to interpret, but can become complex with large datasets.
- Support Vector Machines (SVMs): These algorithms find optimal hyperplanes to separate data into different classes. Effective for high-dimensional data.
- Bayesian Networks: These probabilistic graphical models represent relationships between variables, allowing for probabilistic reasoning under uncertainty.
These algorithms are used extensively in diverse applications—from medical diagnosis (Bayesian networks) to spam filtering (SVMs) and fraud detection (decision trees).
Probabilistic Reasoning
AI often deals with uncertainty. Probabilistic reasoning enables AI systems to make informed decisions even when complete information is lacking. Bayesian inference, for example, updates beliefs about events based on new evidence, allowing AI to adapt and learn from new data.
Limitations of Current AI "Thought"
Despite remarkable progress, current AI systems have significant limitations:
Lack of General Intelligence
Most existing AI exhibits narrow or weak AI, excelling at specific tasks but lacking the general intelligence of humans. Achieving Artificial General Intelligence (AGI)—the ability to perform any intellectual task a human being can—remains a major challenge.
Explainability and Transparency ("Black Box" Problem)
Understanding the internal workings of complex AI systems can be incredibly difficult. This "black box" problem hinders our ability to debug errors, identify biases, and ensure trustworthiness.
Ethical Considerations
The increasing capabilities of AI raise profound ethical concerns:
- Bias and Discrimination: AI systems trained on biased data can perpetuate and amplify societal biases.
- Job Displacement: Automation driven by AI may lead to significant job losses.
- Autonomous Weapons: The development of lethal autonomous weapons systems raises serious ethical and security questions.
Conclusion
Understanding the mechanisms of AI "thought"—the interplay of neural networks, algorithms, and data—is crucial for responsible development and deployment. While AI has made tremendous strides, its current limitations, particularly the lack of general intelligence and the "black box" problem, necessitate further research and careful consideration of ethical implications. Continue your exploration of AI's "thought" processes by delving into [link to relevant resource, e.g., a research paper or course] and discover the cutting edge of artificial intelligence. The future of AI "thinking" is being shaped today, and understanding its foundations is key to navigating this rapidly evolving field.
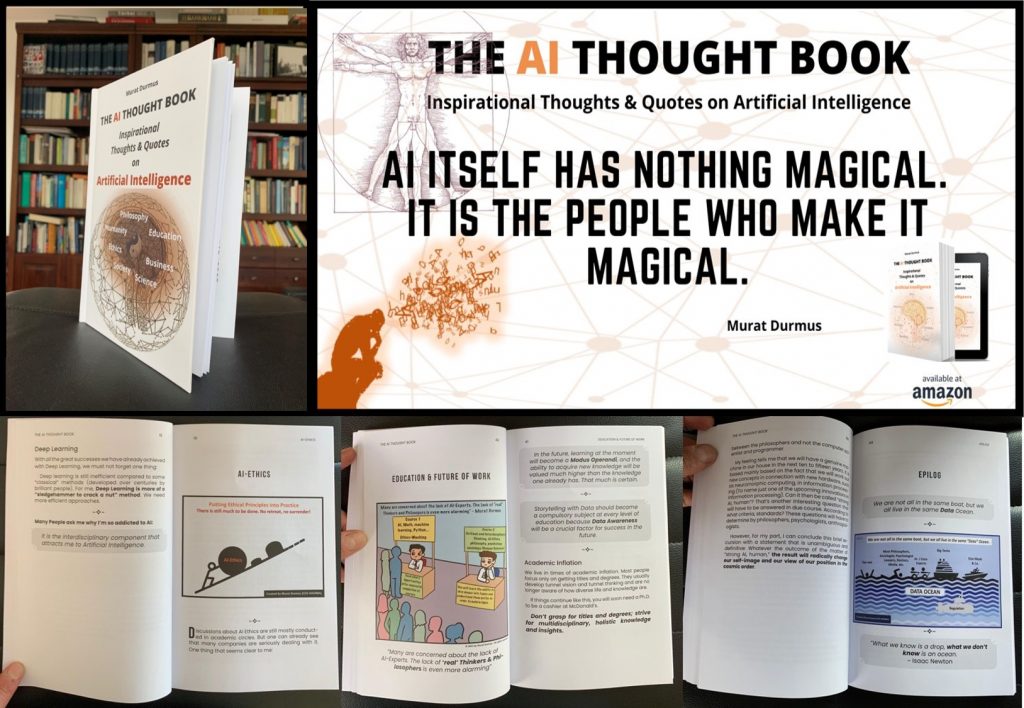
Featured Posts
-
Treasury Market Insights April 8th Events
Apr 29, 2025 -
Senate Majority Leader Schumer I M Staying Put Future Leadership Plans
Apr 29, 2025 -
Jeff Goldblum The Untold Story Behind The Flys Ending
Apr 29, 2025 -
Porsche Macan Electric A Deep Dive Into The New Drive Experience
Apr 29, 2025 -
Safe Driving Practices For Individuals With Adhd A Review Of Current Research
Apr 29, 2025