The Barely-There Thinking Of Artificial Intelligence: A Comprehensive Analysis
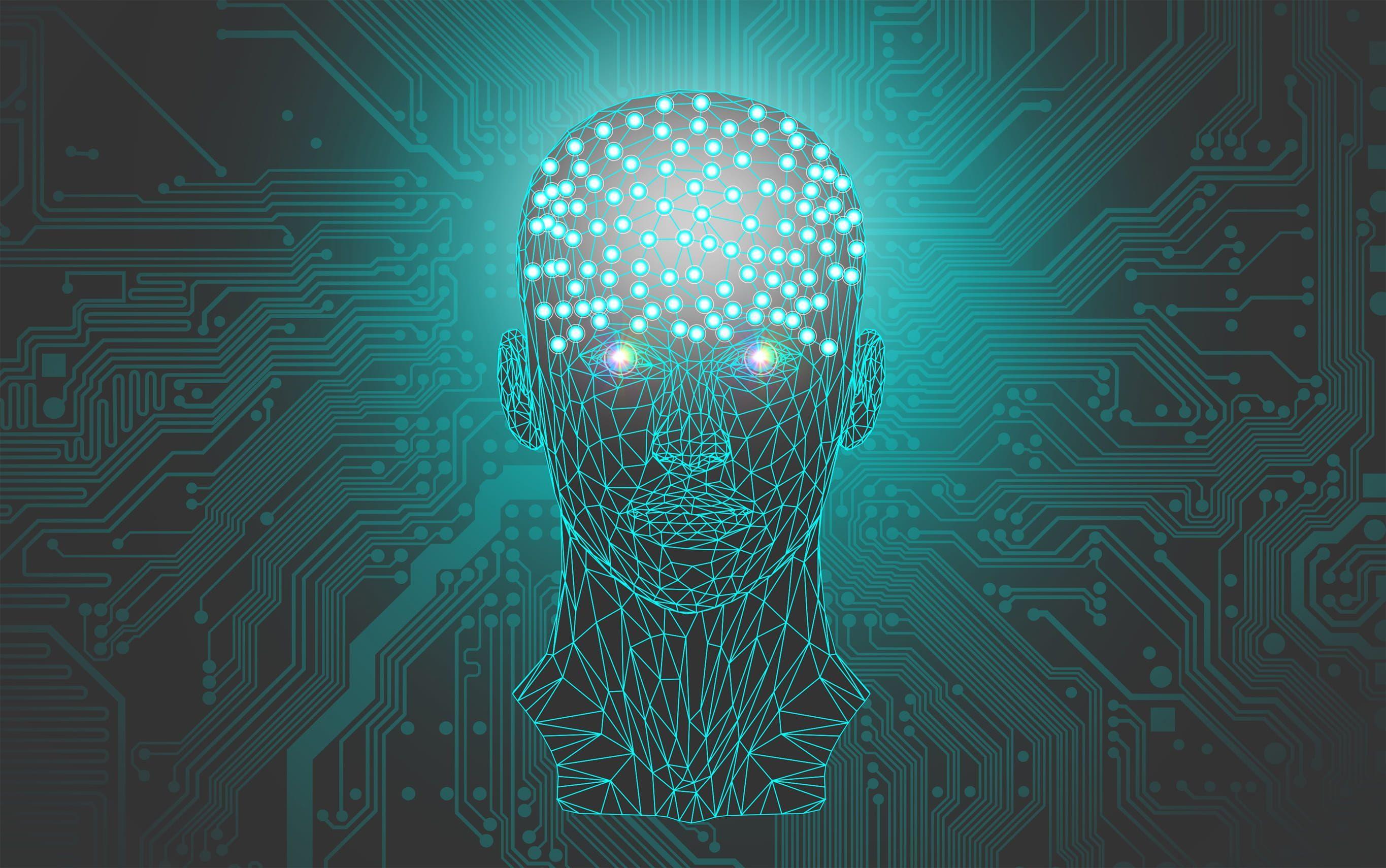
Table of Contents
The Absence of True Understanding in AI
Despite advancements in artificial intelligence, a significant hurdle remains: the absence of true understanding. AI systems often excel at specific tasks but struggle with the nuanced comprehension that comes naturally to humans.
The Problem of Context and Nuance
AI's interpretation of language and situations is often hampered by its inability to grasp context and subtle nuances. This "barely-there" thinking is evident in several ways:
- Misinterpretation of sarcasm and idioms: AI systems frequently fail to recognize sarcasm, interpreting literal meanings even when the context clearly indicates irony or humor. Similarly, idioms and figurative language often lead to misinterpretations.
- Limitations of Natural Language Processing (NLP): Current NLP techniques, while advanced, still struggle with the complexities of human language, including ambiguity, implicit meaning, and emotional undertones. They lack the contextual understanding necessary for truly insightful interpretation.
- Absence of "world knowledge": Unlike humans, most AI systems lack the vast store of general knowledge about the world that informs our understanding. This lack of "world knowledge" significantly limits their ability to interpret information accurately.
The Lack of Common Sense Reasoning
A crucial aspect of human intelligence is common sense reasoning – the ability to make simple inferences and deductions based on everyday knowledge and experience. AI systems, however, often lack these fundamental abilities:
- Failure to make obvious inferences: AI might fail to understand simple cause-and-effect relationships or draw obvious conclusions from a set of facts, a clear example of barely-there thinking.
- Challenges in imbuing common sense: Developing AI systems with common sense reasoning is a major challenge in AI research. It requires moving beyond data-driven approaches and incorporating more sophisticated reasoning mechanisms.
- Narrow AI vs. General AI: The distinction between narrow AI (designed for specific tasks) and general AI (with human-level intelligence) highlights this limitation. Current AI systems are largely narrow AI, lacking the breadth and depth of understanding of general AI.
The Data Dependency and Bias Problem
AI's reliance on data introduces critical limitations, particularly concerning bias and adaptability.
The Influence of Biased Training Data
The data used to train AI systems can contain inherent biases, leading to unfair or discriminatory outcomes. This is a critical aspect of the barely-there thinking in current AI systems.
- Examples of AI bias: AI bias has been observed in facial recognition systems (more accurate for lighter skin tones), loan applications (disadvantageous for certain demographic groups), and criminal justice (predictive policing with racial biases).
- Data diversity and bias mitigation: Addressing AI bias requires careful attention to data diversity, employing bias mitigation techniques during training, and rigorously evaluating AI systems for fairness.
- Ethical implications: The ethical implications of biased AI systems are profound, potentially perpetuating and amplifying existing societal inequalities.
The Limitations of Data-Driven Approaches
AI's data-driven nature limits its ability to handle novel or unexpected situations.
- Incomplete or inconsistent data: AI systems struggle when faced with incomplete, inconsistent, or noisy data. This makes them less robust and adaptable to real-world scenarios.
- Need for adaptable algorithms: More robust and adaptable AI algorithms are needed to address the challenges of incomplete and inconsistent data.
- Out-of-distribution data: The performance of AI systems often degrades significantly when confronted with "out-of-distribution" data – data that differs significantly from the data used during training.
The Implications of Barely-There Thinking in AI
The limitations of current AI systems raise significant concerns.
Safety Concerns and Risk Mitigation
The barely-there thinking of AI poses serious safety risks, especially in critical applications.
- AI failures with real-world consequences: Instances of AI system failures have already resulted in accidents and injuries, highlighting the need for improved safety mechanisms.
- Robust safety mechanisms and testing: Rigorous testing and the implementation of robust safety mechanisms are crucial to mitigate the risks associated with deploying AI in safety-critical applications.
The Future of AI Development
Addressing the limitations of current AI systems requires ongoing research and development.
- Explainable AI (XAI), neuro-symbolic AI, and reinforcement learning: Research areas like XAI (making AI decision-making more transparent), neuro-symbolic AI (combining neural networks with symbolic reasoning), and advanced reinforcement learning techniques hold promise for building more sophisticated AI.
- Hybrid approaches: Hybrid approaches that combine the strengths of AI with human intelligence could help overcome some of the limitations of current AI systems.
Conclusion
The barely-there thinking of artificial intelligence is a central challenge in the field. We've explored how AI struggles with context, common sense, and bias, leading to safety concerns and ethical implications. Understanding these limitations is crucial for responsible AI development. Key takeaways include the importance of addressing bias in training data, developing more robust and adaptable algorithms, and exploring hybrid AI approaches that leverage human expertise. The barely-there thinking of artificial intelligence is a crucial area of ongoing research. Continue exploring this topic to contribute to the responsible development of AI and mitigate potential risks. Let's work together to ensure AI's future benefits outweigh its inherent limitations.
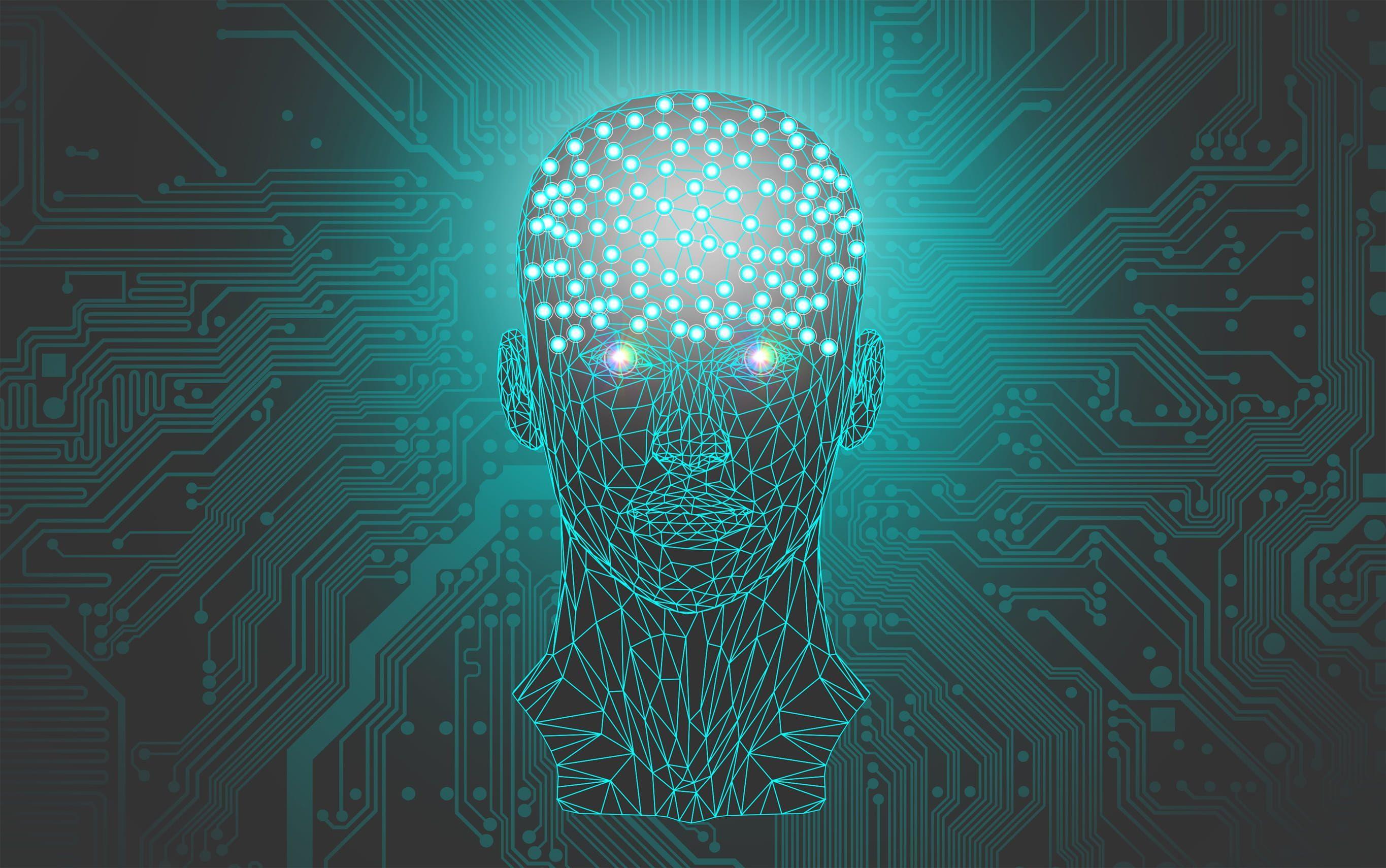
Featured Posts
-
Trump Supporter Ray Epps Defamation Suit Against Fox News Jan 6 Falsehoods Alleged
Apr 29, 2025 -
Analysis Factors That Could Prevent Trumps Tax Bill From Passing
Apr 29, 2025 -
Mlb Recap Twins Top Mets 6 3 In Key Series Matchup
Apr 29, 2025 -
Mine Managers Silence Yukon Politicians Consider Contempt Charges
Apr 29, 2025 -
Analyse Deutsche Teams Im Champions League Vergleich
Apr 29, 2025