The Illusion Of Intelligence: Decoding AI's Limited Thinking Capabilities
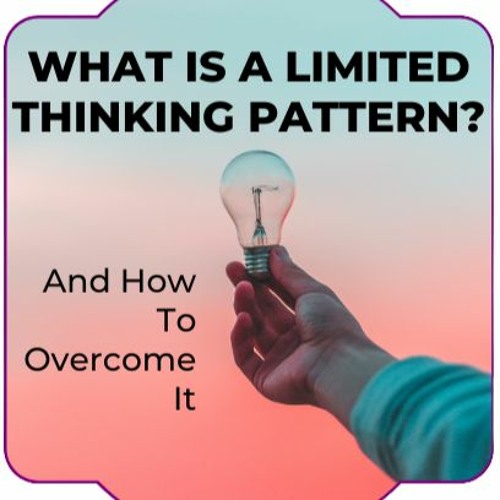
Table of Contents
Artificial intelligence (AI) refers to the simulation of human intelligence processes by machines, especially computer systems. Intelligence, on the other hand, encompasses a complex array of cognitive abilities, including learning, problem-solving, reasoning, and adaptation. This article's purpose is to explore the significant limitations of current AI systems, despite their remarkable achievements in narrow domains. We'll examine how impressive feats of machine learning and deep learning often mask a fundamental lack of genuine cognitive abilities.
AI's Reliance on Data: The Foundation of its "Intelligence"
AI systems, particularly those leveraging machine learning and deep learning, learn through exposure to massive datasets. These systems identify patterns and correlations within the data, allowing them to make predictions and perform tasks with apparent intelligence. However, this data-driven approach has critical limitations. AI's "intelligence" is fundamentally constrained by the quality and quantity of the data it's trained on.
- Over-reliance on correlation versus causation: AI excels at finding correlations in data, but it often struggles to understand the causal relationships between variables. This can lead to inaccurate predictions and flawed conclusions.
- Data bias leading to unfair or inaccurate outputs: If the training data reflects existing societal biases, the AI system will likely perpetuate and even amplify those biases in its outputs. This can have serious consequences in areas like loan applications, criminal justice, and hiring processes.
- The "black box" problem: Many complex AI systems, especially deep learning models, function as "black boxes." It's difficult, if not impossible, to understand the internal processes that lead to a specific output. This lack of transparency hinders our ability to trust and effectively utilize AI in sensitive applications.
- Examples of AI failures: Numerous examples exist where AI systems have failed due to limitations in or biases within their training data. Facial recognition systems showing racial bias, language models generating offensive content, and self-driving cars struggling with unexpected situations are all testaments to this limitation.
The Absence of Common Sense and Contextual Understanding
A hallmark of human intelligence is common sense reasoning – the ability to understand and apply basic knowledge about the world to solve problems and make decisions. Current AI systems largely lack this capability. They struggle with nuanced language, real-world situations, and anything that requires contextual understanding beyond the data they were trained on.
- Inability to interpret sarcasm or humor: AI often fails to grasp the subtle cues that indicate sarcasm or humor, leading to misinterpretations and inappropriate responses.
- Difficulty with abstract concepts and analogies: Abstract reasoning and the ability to form analogies are crucial aspects of human intelligence that remain largely absent in current AI systems.
- Challenges in adapting to unexpected situations or changes in context: AI systems typically perform well within the boundaries of their training data. However, they often struggle when faced with unexpected situations or changes in context that deviate from their learned patterns.
- Examples: Imagine asking an AI to "open the door." A human understands this request within the context of the situation – perhaps needing to find a key or use a handle. An AI, lacking common sense, might simply respond with a literal interpretation, unable to connect the request to the physical act of opening a door.
The Limits of Current AI Architectures: Beyond Narrow Intelligence
Current AI is largely "narrow AI," meaning it excels at specific tasks but lacks the general intelligence of humans. Artificial General Intelligence (AGI), also known as human-level AI, remains a distant goal. Developing AGI presents immense challenges, primarily due to the complexity of human cognition and consciousness.
- Lack of creativity, self-awareness, and emotional intelligence: Current AI systems lack the creativity, self-awareness, and emotional intelligence that are fundamental to human intelligence.
- Ethical implications of advanced AI: The development of more advanced AI systems raises significant ethical concerns, including potential biases, job displacement, and the possibility of autonomous weapons systems.
- Ongoing research and development in AGI: Significant research and development efforts are underway to explore pathways towards AGI, focusing on areas like neuromorphic computing, hybrid AI systems, and advanced machine learning techniques.
Ethical Considerations and the Future of AI
The deployment of AI in critical decision-making processes carries significant ethical implications. Biases embedded in algorithms can lead to unfair or discriminatory outcomes, affecting various communities disproportionately.
- Bias in algorithms: AI algorithms can reflect and amplify existing societal biases, leading to discriminatory outcomes in areas like loan applications, hiring, and criminal justice.
- Job displacement due to automation: The increasing automation of tasks through AI raises concerns about job displacement and the need for workforce retraining and adaptation.
- Transparency and explainability: The need for transparency and explainability in AI systems is paramount to ensuring accountability and building trust. We need to understand how AI arrives at its conclusions, especially in high-stakes scenarios.
- Human oversight: Effective human oversight is crucial in the development and deployment of AI systems to mitigate risks and ensure responsible use.
Understanding the Illusion and Shaping the Future of AI
This article has highlighted key limitations of current AI: its dependence on vast amounts of data, its lack of common sense and contextual understanding, and the significant gap between narrow AI and the elusive goal of AGI. While AI demonstrates impressive computational power, it's crucial to remember that we're still far from achieving true artificial intelligence. The impressive capabilities of AI today should not overshadow its fundamental limitations.
It's vital to understand AI's limitations to avoid over-reliance and misuse. Continue learning about the limitations of artificial intelligence and the ethical considerations surrounding its development. Only through critical thinking and responsible AI development can we harness its power for the benefit of humanity. Let's work together to shape a future where AI augments human capabilities responsibly and ethically.
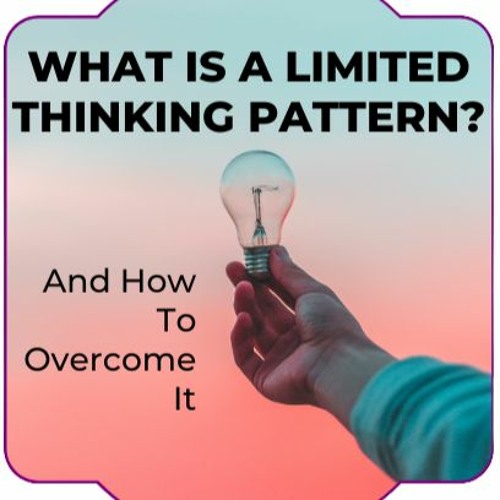
Featured Posts
-
From Street Sweeper To Star The Macario Martinez Story
Apr 29, 2025 -
Double Trouble In Hollywood Writers And Actors Strike Cripples Production
Apr 29, 2025 -
Regulatory Green Light For Hengrui Pharmas Hong Kong Listing
Apr 29, 2025 -
Canada Election Analysis Of Mark Carneys Final Campaign Push
Apr 29, 2025 -
Exclusive Ivy League Schools Create Secret Alliance Against Trump
Apr 29, 2025
Latest Posts
-
Office365 Executive Accounts Targeted In Multi Million Dollar Hacking Scheme
Apr 29, 2025 -
Federal Investigation Hacker Made Millions Targeting Executive Office365 Accounts
Apr 29, 2025 -
Millions Stolen In Exec Office365 Account Compromise Federal Charges Filed
Apr 29, 2025 -
Office365 Intrusion Nets Millions For Hacker Fbi Investigation Reveals
Apr 29, 2025 -
Navigating The Difficulties Of All American Manufacturing
Apr 29, 2025