The Impact Of Outdated Business Applications On AI Initiatives
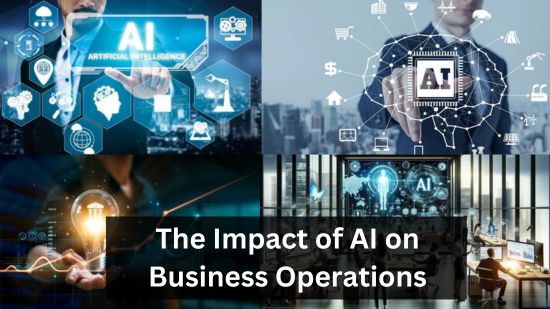
Table of Contents
Data Integration Challenges with Legacy Systems
Outdated business applications frequently present significant hurdles to successful AI implementation, primarily through challenges in data integration. The complexity and cost associated with integrating legacy systems into a modern AI architecture are often underestimated.
Data Silos and Inconsistent Data Formats
Outdated applications often create data silos, making it difficult to consolidate and analyze the data necessary for effective AI model training. This fragmented data landscape significantly impacts the accuracy and reliability of AI insights.
-
Types of Data Silos:
- Departmental Silos: Data is isolated within individual departments (e.g., marketing, sales, finance), hindering cross-functional analysis.
- Application-Specific Silos: Data is trapped within specific applications, preventing seamless data exchange.
- Geographical Silos: Data is scattered across different geographical locations, making consolidation challenging.
-
Consequences of Inconsistent Data Formats: Inconsistent data formats, such as variations in date formats (MM/DD/YYYY vs. DD/MM/YYYY), missing fields, or different data types for the same variable, severely impact AI model accuracy. These inconsistencies lead to errors, biases, and ultimately, unreliable predictions.
-
Data Cleansing and Standardization: Before AI implementation, a thorough data cleansing and standardization process is crucial. This involves identifying and resolving inconsistencies, handling missing values, and transforming data into a consistent format suitable for AI model training. This step is often the most time-consuming but crucial aspect of AI implementation with legacy systems.
Difficulty in Real-time Data Integration
Many legacy systems lack the capabilities for real-time data streaming, crucial for many AI applications. Real-time data is essential for applications requiring immediate responses and dynamic adjustments.
-
Batch Processing vs. Real-time Processing: Batch processing involves processing data in large batches at scheduled intervals, while real-time processing handles data as it arrives, allowing for immediate action.
-
AI Applications Requiring Real-time Data Integration:
- Fraud detection: Real-time analysis of transaction data is essential for identifying fraudulent activities immediately.
- Predictive maintenance: Monitoring sensor data in real-time allows for predictive maintenance of equipment, preventing costly downtime.
- Supply chain optimization: Real-time tracking of inventory and shipments enables efficient supply chain management.
-
Solutions for Real-time Data Integration: Utilizing APIs and ETL (Extract, Transform, Load) tools can bridge the gap between legacy systems and modern AI platforms, facilitating real-time data integration. Cloud-based solutions often provide robust capabilities for real-time data processing.
Limited Scalability and Performance Issues
Outdated infrastructure and a lack of scalability are major roadblocks when integrating legacy systems into AI initiatives. These limitations can significantly hamper the potential of your AI investments.
Outdated Infrastructure and Hardware
Legacy systems might be running on outdated hardware or infrastructure, impacting the performance of AI algorithms which require significant processing power. The computational demands of many AI models necessitate robust infrastructure capable of handling large datasets and complex computations.
-
Hardware Limitations: Outdated hardware can restrict AI model training and deployment, leading to slow processing times and limited model complexity.
-
Bottlenecks and Increased Latency: Processing bottlenecks can emerge, leading to increased latency and delays in AI application responses, ultimately compromising the effectiveness and speed of the AI system.
-
Cloud Computing and Infrastructure Upgrades: Migrating to cloud computing or upgrading on-premise infrastructure can provide the necessary processing power and scalability for AI initiatives. This is often a necessary step in modernizing your technology and allowing seamless AI integration.
Scalability Challenges
Scaling AI initiatives requires adaptable infrastructure; older systems often struggle to handle the increased data volume and computational demands. Growth and scalability are crucial factors to consider when evaluating your legacy systems.
-
Increased User Traffic and Data Volume: Legacy systems might struggle to handle increased user traffic and data volume during AI processing, potentially leading to system overload and performance degradation.
-
System Crashes and Performance Degradation: During peak usage, legacy systems may experience system crashes or significant performance degradation, impacting the availability and reliability of AI applications.
-
Scalable Cloud Solutions and Microservices Architectures: Cloud solutions and microservices architectures offer the flexibility and scalability needed to handle increasing data volumes and user traffic, ensuring robust AI performance.
Security Risks and Compliance Issues
Security vulnerabilities and compliance issues related to outdated business applications pose serious risks to AI initiatives, possibly leading to data breaches and legal repercussions. Modernizing your legacy systems is a critical step in mitigating these risks.
Vulnerabilities in Outdated Systems
Legacy applications often lack the latest security patches and protocols, making them vulnerable to cyberattacks which can compromise sensitive data used for AI. The security risks associated with outdated software are substantial and cannot be ignored.
-
Common Security Vulnerabilities: Outdated systems are susceptible to various vulnerabilities, including SQL injection, cross-site scripting (XSS), and buffer overflow attacks.
-
Data Breaches and Impact on AI Project Security: Data breaches can expose sensitive data used for AI model training, compromising the integrity and security of the AI project. This can also lead to significant financial penalties and reputational damage.
-
Regular Security Audits and Updates: Regular security audits and timely updates are essential for mitigating security risks in outdated systems and ensuring the protection of sensitive data used for AI purposes.
Compliance and Regulatory Challenges
Outdated systems may not comply with current data privacy regulations, hindering the use of data for AI initiatives. Compliance is a crucial element in successfully implementing AI systems.
-
Relevant Data Privacy Regulations: Regulations such as GDPR (General Data Protection Regulation) and CCPA (California Consumer Privacy Act) impose strict requirements on data handling and processing.
-
Non-compliance Penalties and Legal Issues: Non-compliance can lead to significant fines and legal issues, potentially derailing AI projects and damaging an organization’s reputation.
-
Data Governance and Compliance Solutions: Implementing robust data governance and compliance solutions is vital for ensuring that data used for AI initiatives complies with all relevant regulations.
Strategies for Overcoming Challenges: Application Modernization
To successfully integrate AI into your business operations, addressing the challenges posed by outdated business applications is paramount. Application modernization is essential in bridging this gap.
Cloud Migration
Migrating legacy applications to the cloud offers improved scalability, enhanced performance, and robust security features. Cloud migration is often a key step in preparing for AI implementation.
Application Refactoring
Rewriting or restructuring existing applications can significantly improve efficiency and compatibility with modern AI tools. Refactoring can improve code quality, maintainability, and scalability, enabling smoother AI integration.
API Integration
Connecting legacy systems to modern applications through APIs enables data integration and interoperability. APIs facilitate seamless data exchange between systems, ensuring that legacy data can be effectively leveraged for AI purposes.
Conclusion
Outdated business applications pose significant challenges to successful AI initiatives. Data integration difficulties, scalability issues, and security risks can severely limit the effectiveness of AI projects. Ignoring these challenges can lead to wasted investment and missed opportunities.
Don't let outdated business applications hinder your AI journey. Assess your current application landscape, identify outdated systems, and proactively plan for application modernization to unlock the true potential of AI for your organization. Investing in modernization strategies, such as cloud migration and API integration, is key to achieving successful AI initiatives and maximizing the return on your investment in AI technology. Start your digital transformation today and reap the rewards of effective AI implementation.
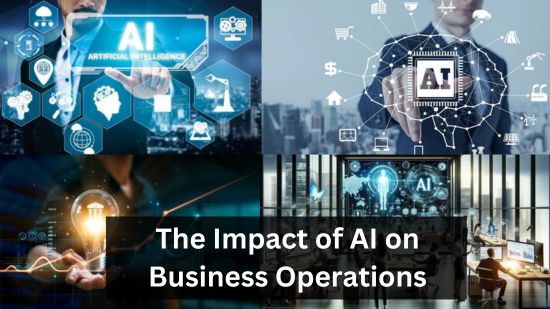
Featured Posts
-
Tongas U 19 Womens Football Team Secures 2025 Ofc Championship Spot
May 01, 2025 -
Stuttgart In Bueyuek Futbol Altyapi Secmeleri Atff Duyurusu
May 01, 2025 -
Polemique Les Celebrations A L Arme A Feu D Une Star De Nba Divisent
May 01, 2025 -
Heartbreak In Home Game Lady Raiders Fall Short Against Cincinnati 56 59
May 01, 2025 -
Plan Your Next Foodie Adventure A Windstar Cruise Experience
May 01, 2025