Turning "Poop" Into Profit: How AI Digests Repetitive Scatological Documents For Podcast Production
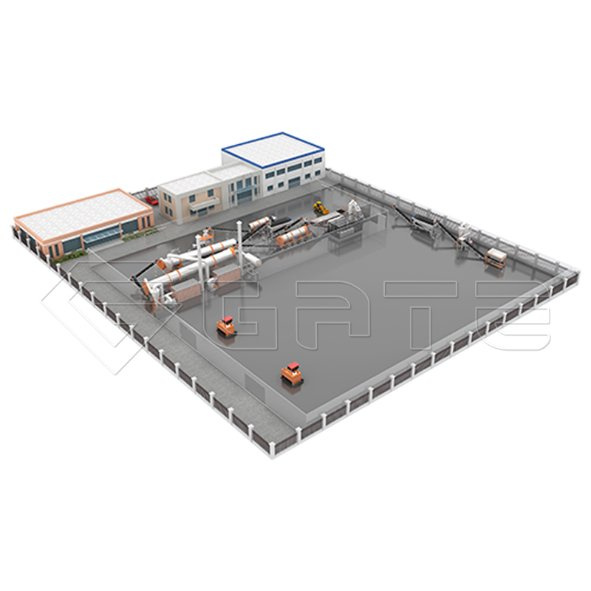
Table of Contents
H2: The Problem of Repetitive Data in Podcast Research
Podcast research often involves reviewing countless documents to uncover crucial information. This manual process presents significant challenges:
H3: Time-Consuming Manual Review: Manually reviewing documents is incredibly time-intensive. Researchers face numerous hurdles:
- Identifying key information across multiple sources: Extracting consistent facts from various documents with differing formats and styles is painstaking.
- Synthesizing data from various formats (PDFs, transcripts, etc.): Converting information from various formats into a usable structure adds significant overhead.
- Dealing with inconsistent terminology and phrasing: The same information might be expressed differently across sources, making comparison difficult.
- High risk of human error and missed information: The sheer volume of data makes it easy to miss crucial details or introduce errors during manual review.
H3: Cost Inefficiencies: The financial implications of manual data processing are substantial:
- High labor costs: Employing researchers to manually review documents is expensive, especially for extensive projects.
- Slow turnaround times: Manual processing significantly delays project timelines, missing crucial release windows and impacting revenue.
- Potential for missed opportunities: Delayed production due to manual data processing can mean missing out on timely news cycles or trending topics.
H2: AI-Powered Solutions for Data Digestion
Fortunately, AI offers powerful solutions to overcome these challenges. Specifically, leveraging AI technologies for podcast research can drastically improve efficiency:
H3: Natural Language Processing (NLP): NLP algorithms are key to efficient data processing. They can:
- Understand context and meaning in unstructured text: NLP goes beyond simple keyword searches, grasping the nuanced meaning within sentences and paragraphs.
- Identify key phrases and concepts related to the podcast topic: NLP can extract relevant information even if the phrasing varies slightly across documents.
- Summarize large amounts of text efficiently: AI can condense extensive documents into concise summaries, highlighting key findings without losing crucial information.
- Extract relevant information from different document formats: NLP handles diverse formats (PDFs, Word docs, transcripts) seamlessly.
H3: Machine Learning for Pattern Recognition: Machine learning (ML) models excel at recognizing patterns and variations in repetitive textual data. This allows for:
- Improved accuracy: ML algorithms learn from the data, continually improving their ability to identify and extract relevant information.
- Increased speed: Automation significantly reduces processing time compared to manual methods.
- Scalability: ML models can easily handle large datasets, making them ideal for large-scale podcast research projects.
H3: Specific AI Tools & Technologies: Several AI tools are specifically designed for data processing. For example, platforms like [insert example of NLP platform with link] offer advanced NLP capabilities, including sentiment analysis and topic modeling, which are extremely valuable for podcast research. Similarly, [insert example of data mining tool with link] helps with the extraction of structured data from unstructured sources, enhancing efficiency and data quality.
H2: Case Studies: Turning Scatological Documents into Podcast Gold
Let's examine how AI transforms repetitive data into valuable podcast content:
H3: Example 1: The Medical Podcast: Imagine a medical podcast aiming to discuss the latest research on a specific condition. Manually reviewing hundreds of research papers would be a monumental task. Using an AI-powered solution, researchers could upload all documents, and the AI would efficiently extract key findings, summarizing complex studies into easily digestible information for the podcast, saving hundreds of hours of research time.
H3: Example 2: The Legal Podcast: A legal podcast covering recent court cases could benefit from AI to summarize lengthy legal documents. AI could quickly identify key legal arguments, judgments, and precedents across multiple cases, saving researchers considerable time and ensuring comprehensive coverage.
3. Conclusion
Manual data processing for podcast research is time-consuming, expensive, and prone to errors. AI-powered solutions, leveraging NLP and machine learning, offer a transformative approach. These technologies dramatically increase efficiency, reduce costs, and improve accuracy, allowing podcasters to focus on content creation instead of tedious data sifting. Stop wasting time sifting through repetitive data – start Turning Poop into Profit with the power of AI! Explore AI-powered tools today and unlock the potential for streamlined and successful podcast production.
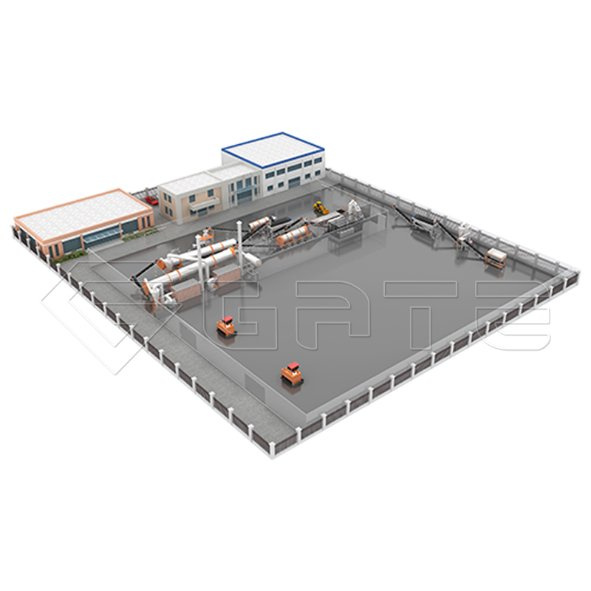
Featured Posts
-
E Ink Spectra
Apr 28, 2025 -
Perplexitys Ceo On The Ai Browser War Taking On Google
Apr 28, 2025 -
High Stock Market Valuations Why Bof A Thinks Investors Shouldnt Panic
Apr 28, 2025 -
New Business Hot Spots Across The Nation An Interactive Map
Apr 28, 2025 -
Boston Red Sox Vs Toronto Blue Jays Lineups Buehler And Outfielder Return
Apr 28, 2025