Understanding AI's "Thought" Processes: A Look At The Current Limitations
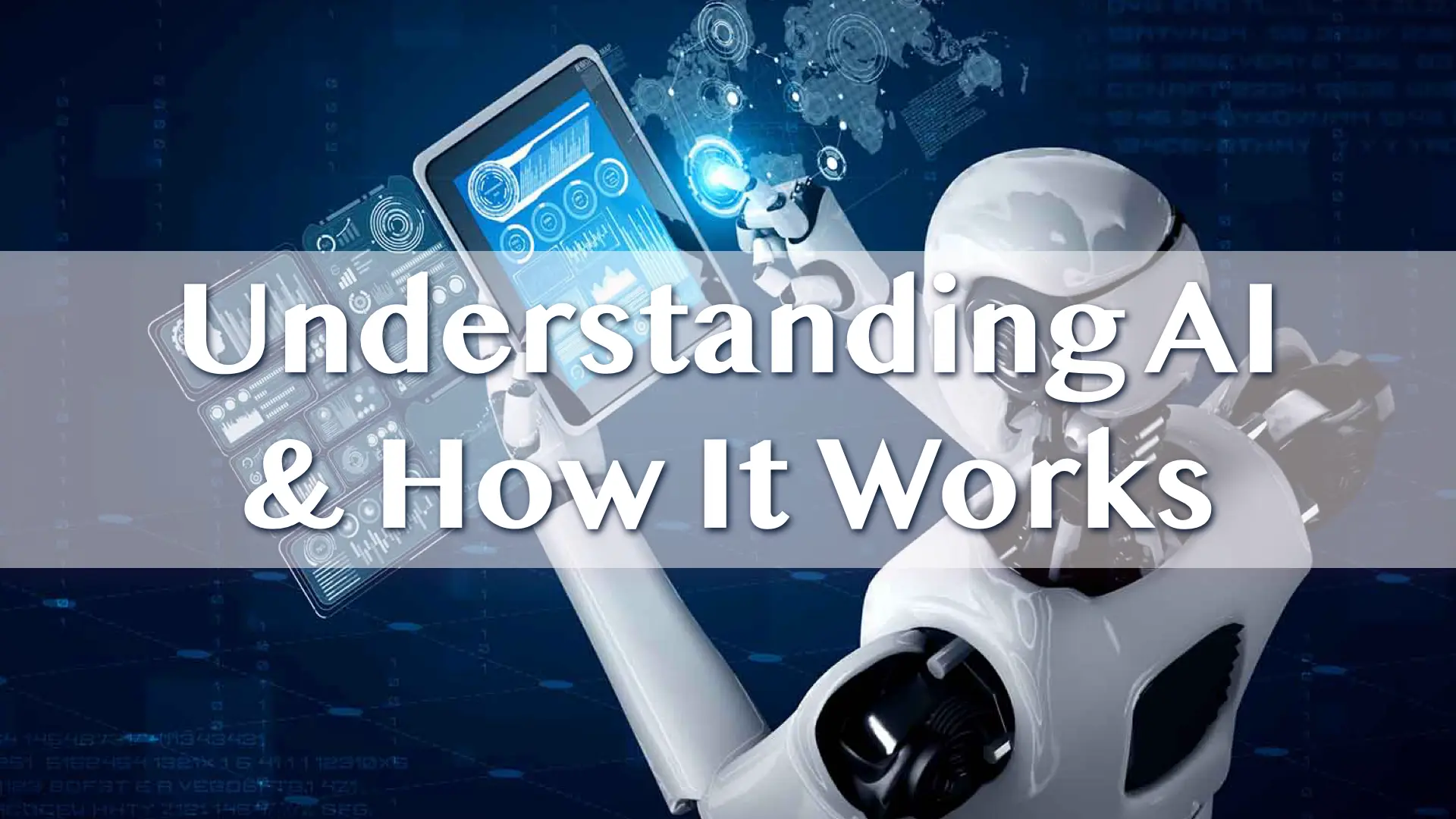
Table of Contents
The Illusion of Understanding: How AI Processes Information
While AI can perform complex tasks, it's crucial to understand that it doesn't "think" like humans. Instead of genuine comprehension, AI uses sophisticated algorithms and vast amounts of data to process information and produce outputs. This fundamental difference shapes its capabilities and limitations.
Machine Learning and Deep Learning: The Engines of AI
AI's apparent intelligence stems largely from machine learning and deep learning. Machine learning involves training algorithms on data to identify patterns and make predictions. Deep learning, a subset of machine learning, uses artificial neural networks with multiple layers to analyze data more abstractly.
- Supervised learning: The algorithm learns from labeled data, where inputs are paired with desired outputs (e.g., training an image recognition system with labeled images of cats and dogs).
- Unsupervised learning: The algorithm identifies patterns in unlabeled data (e.g., clustering customers based on purchasing behavior).
- Reinforcement learning: The algorithm learns through trial and error, receiving rewards or penalties for its actions (e.g., training a robot to navigate a maze).
However, even these powerful techniques have limitations. Overfitting, where the model performs well on training data but poorly on new data, is a common problem. Furthermore, biases present in the training datasets can lead to unfair or inaccurate results in the AI's output. Understanding these limitations is key to responsible AI development.
The Lack of Contextual Understanding
One significant limitation of current AI is its struggle with nuanced language and real-world context. While AI can process vast amounts of text, it often lacks the common sense reasoning and contextual awareness that humans possess.
- AI frequently misinterprets sarcasm, idioms, and complex instructions, leading to unexpected or nonsensical outputs. Consider the challenge of understanding humor or subtle social cues.
- Current Natural Language Processing (NLP) models, while impressive, often struggle with ambiguity and the multifaceted nature of human communication. They lack the ability to truly understand the meaning behind words and sentences in the way humans do. The missing element is common sense reasoning, which allows humans to make inferences and fill in gaps in information.
The Absence of True Consciousness and Self-Awareness
A fundamental difference between AI and human intelligence lies in consciousness and self-awareness. AI, even the most advanced systems, lacks the subjective experience and self-reflection that define human consciousness.
The Turing Test and its Limitations
The Turing Test, a benchmark for AI intelligence, assesses a machine's ability to exhibit intelligent behavior equivalent to, or indistinguishable from, that of a human. However, passing the Turing Test does not equate to possessing consciousness or genuine understanding. It simply measures the ability to mimic human conversation.
The Problem of Sentience and Emotion
Currently, AI lacks the capacity to experience emotions or possess self-awareness. While research in affective computing aims to imbue AI with emotional intelligence, creating truly sentient AI remains a significant scientific and philosophical challenge.
- The ethical considerations surrounding the development of sentient AI are profound, raising questions about rights, responsibilities, and the potential impact on society. Should sentient AI have rights? What safeguards are needed to prevent misuse? These are crucial questions that need careful consideration.
Practical Limitations and Challenges in AI's "Thought" Processes
Beyond the conceptual limitations, practical challenges hinder the development of truly intelligent AI.
Data Dependency and Bias
AI models are heavily reliant on data. Biased or incomplete datasets inevitably lead to biased or inaccurate AI outputs. Ensuring diverse and representative datasets is crucial for mitigating bias and improving AI fairness.
- Examples of AI bias are prevalent in various applications, including facial recognition systems that perform poorly on certain ethnic groups, loan applications algorithms that discriminate against specific demographics, and many more.
Computational Costs and Energy Consumption
Training and deploying advanced AI models require immense computational resources and energy consumption. This has significant environmental implications and poses a barrier to widespread access to advanced AI technologies.
- Strategies for optimizing AI model efficiency, such as developing more efficient algorithms and using specialized hardware, are essential for making AI more sustainable and accessible.
Conclusion
AI's "thought" processes are currently based on sophisticated algorithms and data processing, but they lack true understanding, consciousness, and self-awareness. Current limitations include data dependency, bias, and significant computational costs. Understanding these limitations is critical for responsible AI development. Continued research is vital to address these limitations and harness the full potential of AI safely and ethically. Understanding AI's "thought" processes is crucial for responsible development and deployment. Further research into AI's limitations is essential to harness its power safely and ethically, fostering a future where AI's capabilities are fully understood and utilized. Continue learning about the complexities of AI's thought processes to contribute to a more informed discussion about its future.
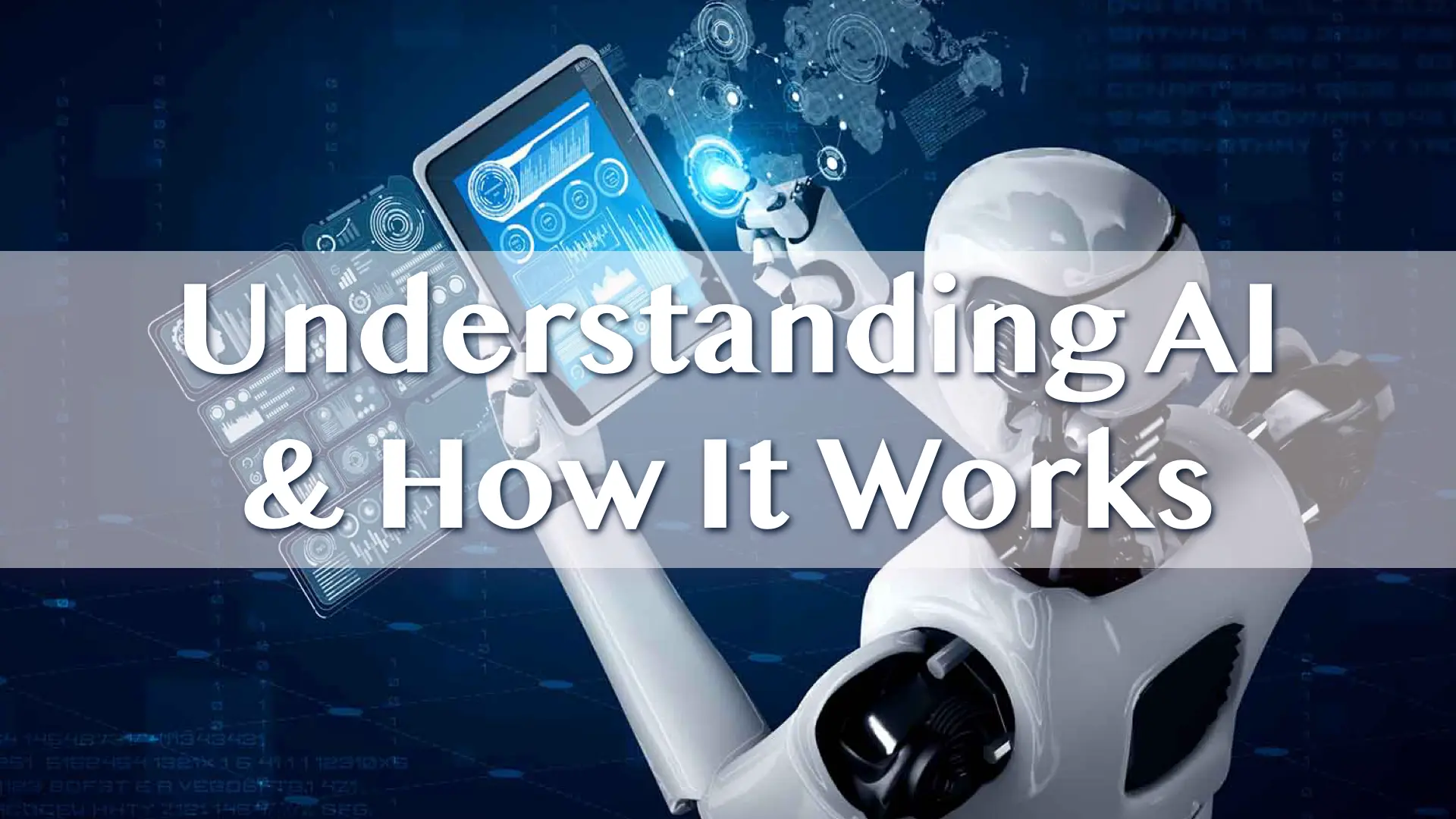
Featured Posts
-
Significant Trans Rights Protest 20 000 Take To The Streets
Apr 29, 2025 -
The Pete Rose Pardon Debate Analyzing Trumps Potential Action And Its Fallout
Apr 29, 2025 -
Mhairi Black On The Misogynistic Underpinnings Of Protecting Women And Girls
Apr 29, 2025 -
Private University Group Challenges Trump Administration Policies
Apr 29, 2025 -
The One Thing Elusive To Iconic Star Jeff Goldblum
Apr 29, 2025