Understanding And Implementing The Updated CNIL Guidelines On AI Models
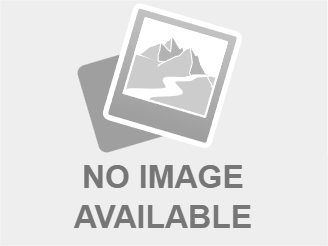
Table of Contents
Key Changes in the Updated CNIL AI Guidelines
The revised CNIL AI Guidelines introduce several key modifications compared to the previous version. These changes reflect a stronger emphasis on data protection by design and algorithmic accountability, demanding a more proactive approach to AI development and deployment. Understanding these changes is crucial for achieving CNIL AI compliance.
-
Increased emphasis on data minimization and purpose limitation in AI development: The updated guidelines strongly reinforce the principles of data minimization and purpose limitation. This means organizations must only collect the minimum necessary data for the specific AI purpose and avoid using it for unintended purposes. Failure to comply could result in significant fines. This necessitates a thorough review of data collection practices and a clear definition of AI objectives. Keywords: CNIL AI compliance, data protection by design.
-
Stronger requirements for transparency and explainability of AI decision-making processes: The CNIL now mandates greater transparency in how AI systems make decisions, particularly those impacting individuals. This involves clearly explaining the logic behind AI-driven outcomes, making the decision-making process more understandable and auditable. Organizations must be able to justify the AI's reasoning and demonstrate its fairness. Keywords: algorithmic accountability, explainable AI (XAI).
-
New guidance on algorithmic impact assessments (AIAs) and their scope: The updated guidelines provide more detailed guidance on conducting AIAs, emphasizing the importance of proactively identifying and mitigating potential risks associated with AI systems. AIAs are no longer optional for high-risk applications; they are a crucial element of CNIL AI compliance. Keywords: AI risk management, CNIL AI best practices.
-
Clarified responsibilities for data controllers and processors regarding AI systems: The CNIL has clarified the responsibilities of both data controllers and processors in ensuring compliance with the updated guidelines. This includes clear lines of accountability for data protection throughout the AI lifecycle. This necessitates a well-defined framework for roles and responsibilities within organizations. Keywords: data controller, data processor, AI governance.
-
Updated recommendations on data security and protection in the context of AI: The guidelines provide updated recommendations on securing AI systems and protecting the data they process. This includes specific considerations for data encryption, access controls, and vulnerability management. Robust security measures are essential to prevent data breaches and comply with CNIL regulations. Keywords: data security, AI security, cybersecurity.
-
Specific guidance on the use of AI in sensitive areas like healthcare and law enforcement: The guidelines offer tailored recommendations for organizations using AI in sensitive sectors, emphasizing the need for particularly rigorous data protection measures and algorithmic fairness assessments. This highlights the increased scrutiny on AI applications involving vulnerable populations. Keywords: AI ethics, responsible AI development.
Practical Steps for Implementing the CNIL AI Guidelines
Implementing the updated CNIL AI Guidelines requires a proactive and comprehensive approach throughout the AI lifecycle. Here are some practical steps to ensure compliance:
Conducting Algorithmic Impact Assessments (AIAs)
AIAs are critical for identifying and mitigating potential risks. The process involves:
- Defining the scope of the AIA: Clearly outline the AI system, its purpose, the data it processes, and the individuals affected.
- Identifying potential risks and vulnerabilities: Analyze the system for biases, discrimination, and other potential harms.
- Implementing appropriate mitigation strategies: Develop and implement measures to reduce or eliminate identified risks.
- Documenting the entire AIA process: Maintain thorough records of the AIA, including findings, mitigation strategies, and ongoing monitoring.
Ensuring Data Minimization and Purpose Limitation
Data minimization and purpose limitation are fundamental principles of the CNIL AI Guidelines.
- Strategies for data minimization during AI model development: Use only the data absolutely necessary for the intended AI purpose.
- Techniques for ensuring purpose limitation is respected: Clearly define the purpose of data collection and avoid using data for unintended purposes.
- Methods for complying with data subject rights in the context of AI: Ensure individuals can exercise their rights (access, rectification, erasure) regarding data used by AI systems.
Enhancing Transparency and Explainability
Transparency and explainability are key to building trust and ensuring accountability.
- Techniques for explaining how AI systems work: Use clear and understandable language to explain the AI's functioning to users.
- Methods for providing users with information about the use of their data: Inform users about how their data is collected, used, and protected by the AI system.
- Strategies for addressing potential biases in AI systems: Implement methods to detect and mitigate biases in AI algorithms and datasets.
Conclusion
The updated CNIL AI Guidelines represent a significant step towards responsible AI development in France. By understanding and diligently implementing these guidelines—including conducting thorough AIAs, minimizing data usage, and ensuring transparency—organizations can ensure compliance, mitigate risks, and build trust with their users. Ignoring these regulations could lead to severe penalties. Therefore, proactive engagement with the CNIL AI Guidelines is paramount. Start your journey towards CNIL AI compliance today by reviewing the detailed requirements and implementing the practical steps outlined in this article. Remember, staying informed about updates to the CNIL AI Guidelines is critical for ongoing compliance.
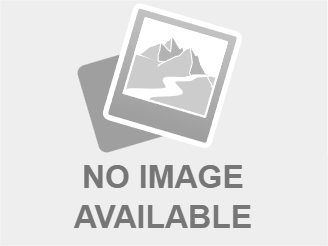
Featured Posts
-
Eskisehir De Tip Egitimi Ve Stres Yoenetimi Boksun Faydalari
Apr 30, 2025 -
New Cnil Ai Guidelines Key Changes And Compliance Strategies
Apr 30, 2025 -
Understanding Beyonce And Jay Zs Decision To Shield Sir Carter
Apr 30, 2025 -
Increased Data Center Demand Drives Schneider Electrics Positive 2024 Financial Projections
Apr 30, 2025 -
Noa Argamani Included In Times 100 Most Influential People 2025 List
Apr 30, 2025