We Now Know How AI "Thinks"—and It's Barely Thinking At All
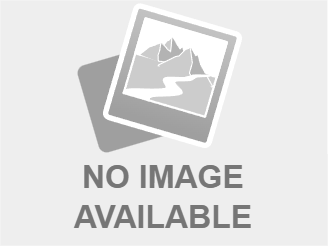
Table of Contents
The Illusion of Intelligence: Understanding AI's Capabilities and Limitations
The term "AI thinking" itself is misleading. Current AI systems are far from possessing the consciousness or general intelligence often portrayed in popular culture. To understand this, we must differentiate between two types of AI:
Narrow AI vs. General AI
- Narrow AI: This is the type of AI we have today. It's designed to excel at a specific task, often performing it better than humans. Examples include:
- Image recognition: Identifying objects, faces, and scenes in images.
- Language translation: Converting text or speech from one language to another.
- Game playing: Mastering complex games like chess or Go.
- General AI: This is hypothetical AI with human-level intelligence, capable of understanding, learning, and applying knowledge across a wide range of tasks. It's the stuff of science fiction, and we're far from achieving it.
This distinction is crucial. Current AI triumphs are impressive feats of narrow AI, not evidence of genuine general intelligence.
Statistical Pattern Recognition, Not True Understanding
At its core, most AI, especially machine learning models, functions through statistical pattern recognition. These systems identify patterns in massive datasets and use these patterns to make predictions or decisions. However, this process is not equivalent to human understanding or reasoning.
- Consider a chatbot that generates grammatically correct responses but lacks genuine comprehension of the conversation's meaning. It manipulates words based on statistical probabilities, not semantic understanding.
- An AI might flawlessly classify images of cats, yet it lacks the intuitive understanding of what constitutes a "cat." It identifies patterns of pixels, not the underlying concept.
The "Black Box" Problem and Explainability in AI
Many complex AI models, particularly deep learning systems, operate as "black boxes." Their decision-making processes are opaque, making it difficult to understand why they arrive at a particular outcome.
- This lack of transparency poses significant challenges:
- Bias: Biased training data can lead to biased outputs, perpetuating societal inequalities.
- Fairness: Unfair or discriminatory outcomes can arise from AI systems whose decision-making processes are not understood.
- Accountability: Determining responsibility for errors or harmful actions becomes difficult when the system's logic is obscured.
Explainable AI (XAI) aims to address this problem, but it's still an active area of research with inherent limitations.
How AI "Learns": A Look Under the Hood
To understand AI's limitations, we need to examine its learning process.
The Role of Data in AI Development
AI development hinges on vast amounts of data used for training. The quality and characteristics of this data profoundly impact the AI's performance and potential biases.
- Data collection methods: The way data is collected can introduce biases from the outset.
- Data cleaning: Removing inaccuracies and inconsistencies is crucial but challenging, particularly with massive datasets.
- Biased datasets: If the training data reflects existing societal biases, the AI will likely perpetuate and even amplify these biases.
Algorithms and Models: The Mechanics of AI "Thinking"
AI utilizes various algorithms to process data and learn patterns. Neural networks, for example, consist of interconnected nodes that process information in layers, mimicking (very loosely) the structure of the human brain. Decision trees, on the other hand, use a branching structure to make decisions based on a series of conditions.
- These algorithms, however sophisticated, perform computations based on mathematical formulas and statistical relationships. They don't possess the qualitative reasoning abilities of a human.
The Implications of AI's "Barely Thinking" Nature
The fact that AI doesn't truly "think" has profound implications.
Ethical Considerations and Societal Impact
Relying on AI systems for critical decisions without fully understanding their limitations raises ethical concerns.
- Autonomous driving: Accidents involving self-driving cars highlight the challenges of ensuring safety and accountability.
- Healthcare diagnostics: AI-driven diagnoses must be carefully reviewed by human experts to minimize errors.
- Criminal justice: AI tools used in risk assessment or sentencing must be carefully evaluated to avoid bias and ensure fairness.
The Future of AI Research and Development
Research continues to push the boundaries of AI, focusing on developing more sophisticated and explainable systems.
- Reinforcement learning: Allows AI to learn through trial and error, improving its performance over time.
- Transfer learning: Enables AI to apply knowledge gained in one domain to new domains, enhancing its adaptability.
- Improving AI robustness and generalizability are crucial research goals, aiming to create more reliable and less error-prone AI systems.
Conclusion
While AI demonstrates impressive capabilities in narrow domains, its "thinking" is fundamentally different from human cognition. It excels at pattern recognition but lacks true understanding and consciousness. Understanding how AI truly thinks requires a balanced perspective, acknowledging both its potential and limitations. The critical evaluation of AI capabilities and responsible AI development are essential to harnessing AI's benefits while mitigating potential risks. Let's work towards a future where AI development prioritizes ethical considerations and societal well-being, ensuring that AI serves humanity effectively and responsibly.
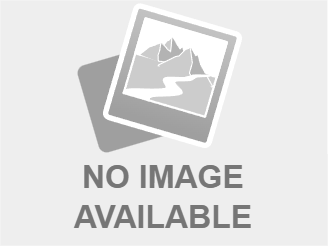
Featured Posts
-
Getting Tickets To The Capital Summertime Ball 2025 Tips And Tricks
Apr 29, 2025 -
Cardinals Conviction And Papal Conclave Voting Eligibility
Apr 29, 2025 -
Essential Willie Nelson Fast Facts For Fans
Apr 29, 2025 -
10 New Nuclear Reactors Approved In China Boosting Energy Capacity
Apr 29, 2025 -
Fealyat Fn Abwzby Tbda 19 Nwfmbr
Apr 29, 2025